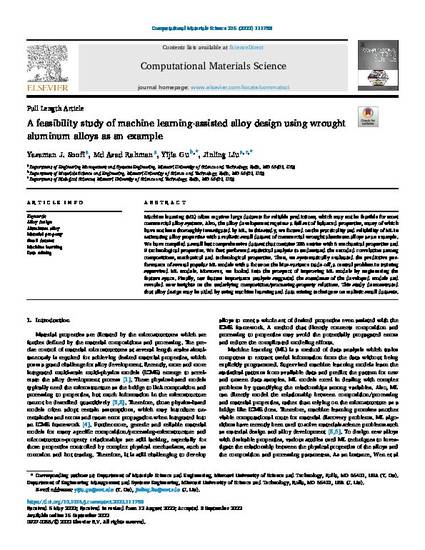
Article
A feasibility study of machine learning-assisted alloy design using wrought aluminum alloys as an example
Computational Materials Science
(2022)
Abstract
Machine learning (ML) often requires large datasets for reliable predictions, which may not be feasible for most commercial alloy systems. Also, the alloy development requires a full set of balanced properties, many of which have not been thoroughly investigated by ML. In this study, we focused on the practicality and reliability of ML in estimating alloy properties with a realistic small dataset of commercial wrought aluminum alloys as an example. We have compiled a small but comprehensive dataset that contains 236 entries with 6 mechanical properties and 9 technological properties. We first performed statistical analysis to understand the encoded correlation among compositions, mechanical and technological properties. Then, we systematically evaluated the predictive performance of several popular ML models with a focus on the bias-variance trade-off, a central problem in training supervised ML models. Moreover, we looked into the prospect of improving ML models by engineering the feature space. Finally, our feature importance analysis suggested the soundness of the developed models and revealed new insights on the underlying composition/processing-property relations. This study demonstrated that alloy design may be aided by using machine learning and data mining techniques on realistic small datasets.
Keywords
- Alloy design,
- Aliminum alloy,
- Material property,
- Small dataset,
- Machine learning,
- Data mining
Disciplines
Publication Date
2022
DOI
10.1016/j.commatsci.2022.111783
Citation Information
Yasaman J Soofi, Md Asad Rahman, Yijia Gu and Jinling Liu. "A feasibility study of machine learning-assisted alloy design using wrought aluminum alloys as an example" Computational Materials Science Vol. 215 (2022) p. 111783 ISSN: 09270256 Available at: http://works.bepress.com/yijia-gu/73/