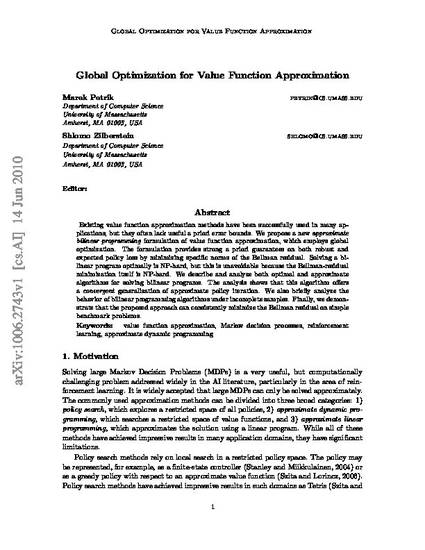
Unpublished Paper
Global Optimization for Value Function Approximation
(2010)
Abstract
Existing value function approximation methods have been successfully used in many applications, but they often lack useful a priori error bounds. We propose a new approximate bilinear programming formulation of value function approximation, which employs global optimization. The formulation provides strong a priori guarantees on both robust and expected policy loss by minimizing specific norms of the Bellman residual. Solving a bilinear program optimally is NP-hard, but this is unavoidable because the Bellman-residual minimization itself is NP-hard. We describe and analyze both optimal and approximate algorithms for solving bilinear programs. The analysis shows that this algorithm offers a convergent generalization of approximate policy iteration. We also briefly analyze the behavior of bilinear programming algorithms under incomplete samples. Finally, we demonstrate that the proposed approach can consistently minimize the Bellman residual on simple benchmark problems.
Keywords
- value function approximation,
- Markov decision processes,
- reinforcement learning,
- approximate dynamic programming
Disciplines
Publication Date
June 14, 2010
Comments
This is the pre-published version harvested from arXiv.
Citation Information
Marek Petrik and Shlomo Zilberstein. "Global Optimization for Value Function Approximation" (2010) Available at: http://works.bepress.com/shlomo_zilberstein/4/