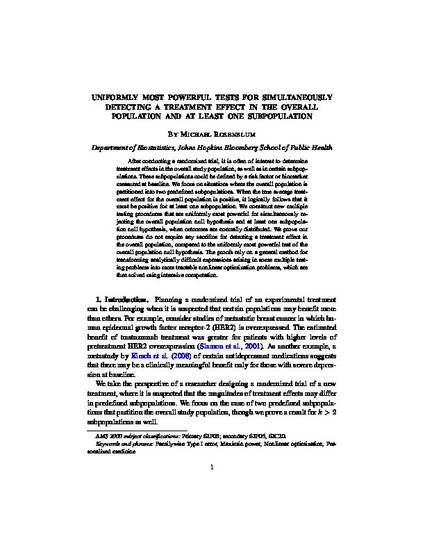
Article
UNIFORMLY MOST POWERFUL TESTS FOR SIMULTANEOUSLY DETECTING A TREATMENT EFFECT IN THE OVERALL POPULATION AND AT LEAST ONE SUBPOPULATION
Johns Hopkins University, Dept. of Biostatistics Working Papers
Date of this Version
6-25-2013
Abstract
After conducting a randomized trial, it is often of interest to determine treatment effects in the overall study population, as well as in certain subpopulations. These subpopulations could be defined by a risk factor or biomarker measured at baseline. We focus on situations where the overall population is partitioned into two predefined subpopulations. When the true average treatment effect for the overall population is positive, it logically follows that it must be positive for at least one subpopulation. We construct new multiple testing procedures that are uniformly most powerful for simultaneously rejecting the overall population null hypothesis and at least one subpopulation null hypothesis, when outcomes are normally distributed. We prove our procedures do not require any sacrifice for detecting a treatment effect in the overall population, compared to the uniformly most powerful test of the overall population null hypothesis. The proofs rely on a general method for transforming analytically difficult expressions arising in some multiple testing problems into more tractable nonlinear optimization problems, which are then solved using intensive computation.
Disciplines
Citation Information
Michael Rosenblum. "UNIFORMLY MOST POWERFUL TESTS FOR SIMULTANEOUSLY DETECTING A TREATMENT EFFECT IN THE OVERALL POPULATION AND AT LEAST ONE SUBPOPULATION" (2013) Available at: http://works.bepress.com/michael_rosenblum/73/