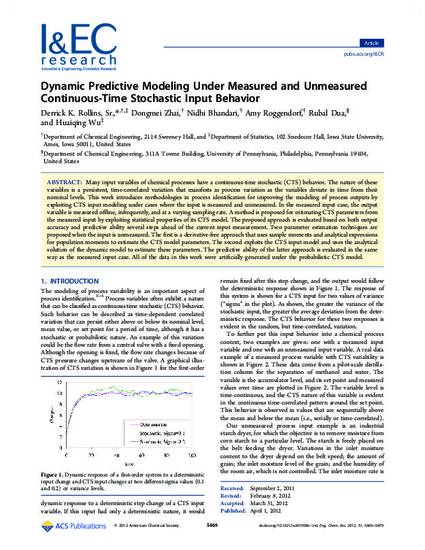
Many input variables of chemical processes have a continuous-time stochastic (CTS) behavior. The nature of these variables is a persistent, time-correlated variation that manifests as process variation as the variables deviate in time from their nominal levels. This work introduces methodologies in process identification for improving the modeling of process outputs by exploiting CTS input modeling under cases where the input is measured and unmeasured. In the measured input case, the output variable is measured offline, infrequently, and at a varying sampling rate. A method is proposed for estimating CTS parameters from the measured input by exploiting statistical properties of its CTS model. The proposed approach is evaluated based on both output accuracy and predictive ability several steps ahead of the current input measurement. Two parameter estimation techniques are proposed when the input is unmeasured. The first is a derivative-free approach that uses sample moments and analytical expressions for population moments to estimate the CTS model parameters. The second exploits the CTS input model and uses the analytical solution of the dynamic model to estimate these parameters. The predictive ability of the latter approach is evaluated in the same way as the measured input case. All of the data in this work were artificially generated under the probabilistic CTS model.
Available at: http://works.bepress.com/derrickk_rollins/11/
Reprinted (adapted) with permission from Industrial and Engineering Chemistry Research 51 (2012): 5469, doi: 10.1021/ie201998b. Copyright 2012 American Chemical Society.