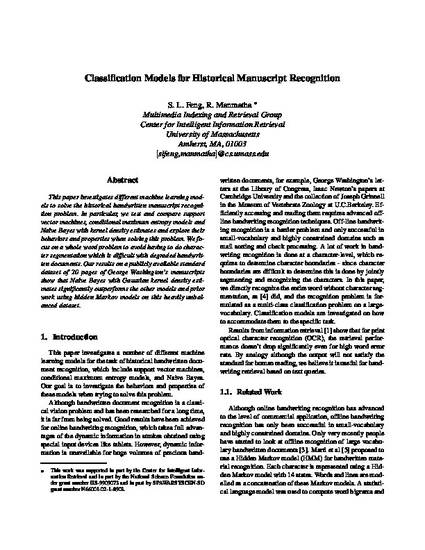
This paper investigates different machine learning models to solve the historical handwritten manuscript recognition problem. In particular, we test and compare support vector machines, conditional maximum entropy models and Naive Bayes with kernel density estimates and explore their behaviors and properties when solving this problem. We focus on a whole word problem to avoid having to do character segmentation which is difficult with degraded handwritten documents. Our results on a publicly available standard dataset of 20 pages of George Washington's manuscripts show that Naive Bayes with Gaussian kernel density estimates significantly outperforms the other models and prior work using hidden Markov models on this heavily unbalanced dataset.
Available at: http://works.bepress.com/r_manmatha/37/