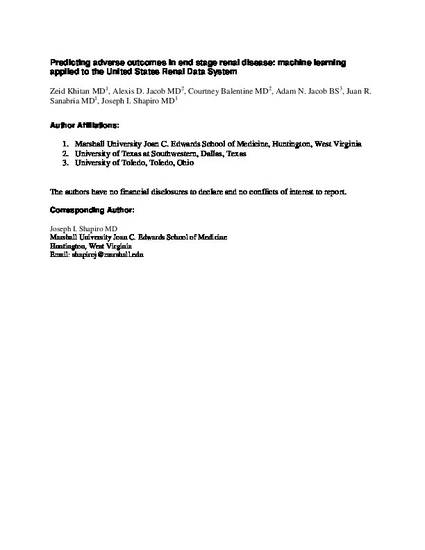
Article
Predicting Adverse Outcomes in End Stage Renal Disease: Machine Learning Applied to the United States Renal Data System
Marshall Journal of Medicine
Keywords
- machine learning,
- end stage renal disease,
- mortality,
- hemodialysis
Disciplines
Abstract
We examined machine learning methods to predict death within six months using data derived from the United States Renal Data System (USRDS). We specifically evaluated a generalized linear model, a support vector machine, a decision tree and a random forest evaluated within the context of K-10 fold validation using the CARET package available within the open source architecture R program. We compared these models with the feed forward neural network strategy that we previously reported on with this data set.
Citation Information
Zeid Khitan, Alexis D Jacob, Courtney Balentine, Adam N Jacob, et al.. "Predicting Adverse Outcomes in End Stage Renal Disease: Machine Learning Applied to the United States Renal Data System" p. 75 Available at: http://works.bepress.com/zeid_khitan/16/