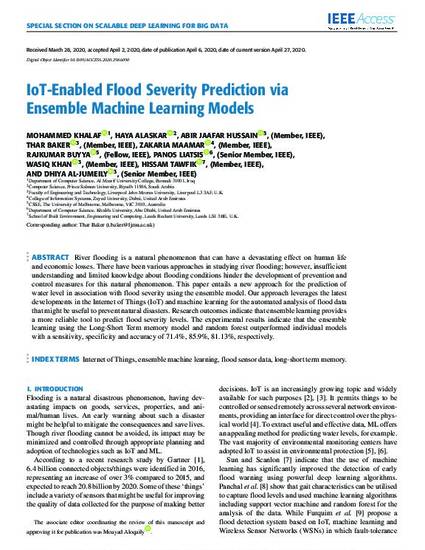
© 2013 IEEE. River flooding is a natural phenomenon that can have a devastating effect on human life and economic losses. There have been various approaches in studying river flooding; however, insufficient understanding and limited knowledge about flooding conditions hinder the development of prevention and control measures for this natural phenomenon. This paper entails a new approach for the prediction of water level in association with flood severity using the ensemble model. Our approach leverages the latest developments in the Internet of Things (IoT) and machine learning for the automated analysis of flood data that might be useful to prevent natural disasters. Research outcomes indicate that ensemble learning provides a more reliable tool to predict flood severity levels. The experimental results indicate that the ensemble learning using the Long-Short Term memory model and random forest outperformed individual models with a sensitivity, specificity and accuracy of 71.4%, 85.9%, 81.13%, respectively.
- ensemble machine learning,
- flood sensor data,
- Internet of Things,
- long-short term memory
Available at: http://works.bepress.com/zakaria-maamar/398/