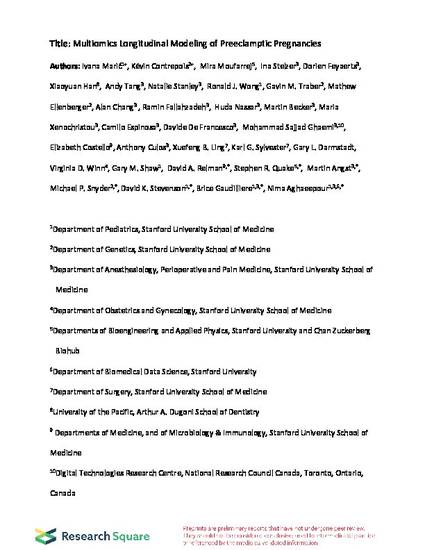
Preeclampsia is a complex disease of pregnancy whose physiopathology remains unclear and that poses a threat to both mothers and infants. Specific complex changes in women's physiology precede a diagnosis of preeclampsia. Understanding multiple aspects of such a complex changes at different levels of biology, can be enabled by simultaneous application of multiple assays. We developed prediction models for preeclampsia risk by analyzing six omics datasets from a longitudinal cohort of pregnant women. A machine learning-based multiomics model had high accuracy (area under the receiver operating characteristics curve (AUC) of 0.94, 95% confidence intervals (CI):[0.90, 0.99]). A prediction model using only ten urine metabolites provided an accuracy of the whole metabolomic dataset and was validated using an independent cohort of 16 women (AUC= 0.87, 95% CI:[0.76, 0.99]). Integration with clinical variables further improved prediction accuracy of the urine metabolome model (AUC= 0.90, 95% CI:[0.80, 0.99], urine metabolome, validated). We identified several biological pathways to be associated with preeclampsia. The findings derived from models were integrated with immune system cytometry data, confirming known physiological alterations associated with preeclampsia and suggesting novel associations between the immune and proteomic dynamics. While further validation in larger populations is necessary, these encouraging results will serve as a basis for a simple, early diagnostic test for preeclampsia.
Available at: http://works.bepress.com/xiaoyuan-han/53/
This is an unpublished pre-print that has not undergone peer review. It should not be considered conclusive, used to inform clinical practice, or referenced by the media as validated information.