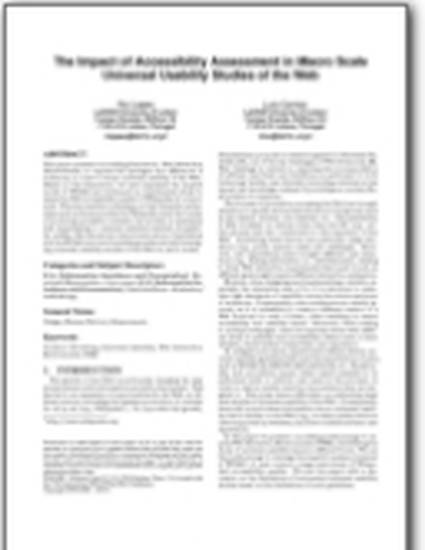
Article
Clustering Mixed Numerical and Low Quality Categorical Data: Significance Metrics on a Yeast Example
IQIS '05 Proceedings of the 2nd international workshop on Information quality in information systems
(2005)
Abstract
We present the M-BILCOM algorithm for clustering mixed numerical and categorical data sets, in which the categorical attribute values (CAs) are not certain to be correct and have associated confidence values (CVs) from 0.0 to 1.0 to represent their certainty of correctness. M-BILCOM performs bi-level clustering of mixed data sets resembling a Bayesian process. We have applied M-BILCOM to yeast data sets in which the CAs were perturbed randomly and CVs were assigned indicating the confidence of correctness of the CAs. On such mixed data sets M-BILCOM outperforms other clustering algorithms, such as AutoClass. We have applied M-BILCOM to real numerical data sets from gene expression studies on yeast, incorporating CAs representing Gene Ontology annotations on the genes and CVs representing Gene Ontology Evidence Codes on the CAs. We apply novel significance metrics to the CAs in resulting clusters, to extract the most significant CAs based on their frequencies and their CVs in the cluster. For genomic data sets, we use the most significant CAs in a cluster to predict gene function.
Disciplines
Publication Date
June 17, 2005
DOI
10.1145/1077501.1077517
Publisher Statement
SJSU users: use the following link to login and access the article via SJSU databases.
Citation Information
Bill Andreopoulos, Aijun An and Xiaogang Wang. "Clustering Mixed Numerical and Low Quality Categorical Data: Significance Metrics on a Yeast Example" IQIS '05 Proceedings of the 2nd international workshop on Information quality in information systems (2005) p. 87 - 98 Available at: http://works.bepress.com/william-andreopoulos/31/