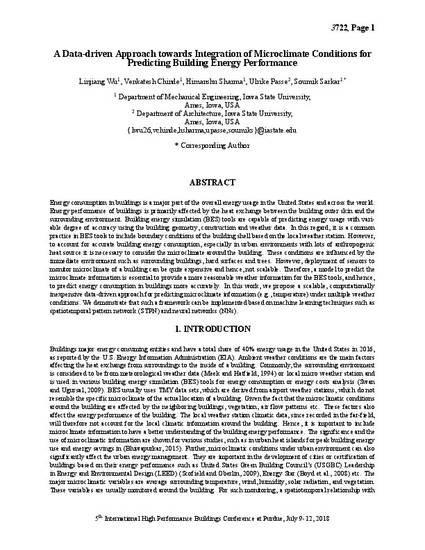
- Building Microclimate prediction,
- Data-driven modeling,
- Spatiotemporal pattern network
Energy consumption in buildings is a major part of the overall energy usage in the United States and across the world. Energy performance of buildings is primarily affected by the heat exchange with the building outer skin and the surrounding environment. Building energy simulation (BES) tools are capable of predicting energy usage with variable degree of accuracy using the building geometry, construction information and weather data. In this regard, it is a common practice in BES tools to include boundary conditions of the building shell based on the local weather station. However, to account for accurate building energy consumption, especially in urban environments with significant amount of anthropogenic heat source, it is necessary to consider the microclimate around the building. These conditions are influenced by the immediate environment such as surrounding buildings, hard surfaces and trees. However, deployment of sensors to monitor microclimate of a building can be quite expensive and hence, not scalable. Therefore, a model to predict the microclimate information based on local weather station is essential to provide a more reasonable outdoor weather information for the BES tools, and hence predicting energy consumption in buildings more accurately. In this work, we propose a scalable, computationally inexpensive data-driven approach for predicting microclimate information (e.g., temperature) under multiple weather conditions. We demonstrate that such a framework can be implemented based on machine learning techniques such as spatiotemporal pattern network (STPN) and neural networks (NNs). We demonstrate the efficacy of our proposed framework by using the predicted microclimate data to predict the building energy consumption with higher accuracy compared to the prediction using local weather station data alone.
Available at: http://works.bepress.com/ulrike_passe/22/