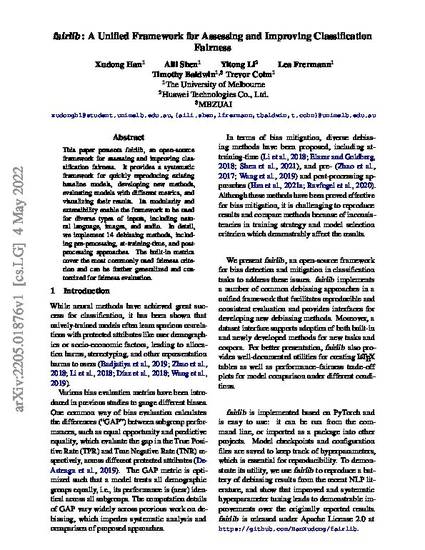
Article
fairlib: A Unified Framework for Assessing and Improving Classification Fairness
arXiv
Document Type
Article
Abstract
This paper presents fairlib, an open-source framework for assessing and improving classification fairness. It provides a systematic framework for quickly reproducing existing baseline models, developing new methods, evaluating models with different metrics, and visualizing their results. Its modularity and extensibility enable the framework to be used for diverse types of inputs, including natural language, images, and audio. In detail, we implement 14 debiasing methods, including pre-processing, at-training-time, and post-processing approaches. The built-in metrics cover the most commonly used fairness criterion and can be further generalized and customized for fairness evaluation. Copyright © 2022, The Authors. All rights reserved.
DOI
10.48550/arXiv.2205.01876
Publication Date
4-3-2022
Keywords
- Baseline models; De-biasing; Evaluating models; Natural languages; Open source frameworks; Pre-processing; Systematic framework; Time processing; Training time; Unified framework; Machine learning; Artificial Intelligence (cs.AI); Computers and Society (cs.CY); Machine Learning (cs.LG)
Disciplines
Citation Information
X. Han, A. Shen, Y. Li, L. Frermann, and T. Baldwin, "fairlib: A Unified Framework for Assessing and Improving Classification Fairness", 2022, arXiv.2205.01876
IR Deposit conditions: non-described
Preprint available on arXiv