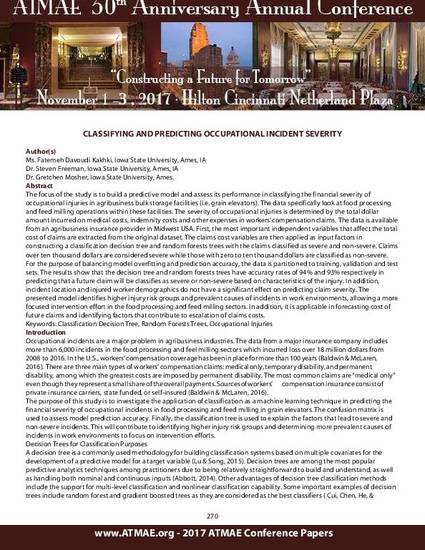
The focus of the study is to build a predictive model and assess its performance in classifying the financial severity of occupational injuries in agribusiness bulk storage facilities (i.e. grain elevators). The data specifically look at food processing and feed milling operations within these facilities. The severity of occupational injuries is determined by the total dollar amount incurred on medical costs, indemnity costs and other expenses in workers’ compensation claims. The data is available from an agribusiness insurance provider in Midwest USA. First, the most important independent variables that affect the total cost of claims are extracted from the original dataset. The claims cost variables are then applied as input factors in constructing a classification decision tree and random forests trees with the claims classified as severe and non-severe. Claims over ten thousand dollars are considered severe while those with zero to ten thousand dollars are classified as non-severe. For the purpose of balancing model overfitting and prediction accuracy, the data is partitioned to training, validation and test sets. The results show that the decision tree and random forests trees have accuracy rates of 94% and 93% respectively in predicting that a future claim will be classifies as severe or non-severe based on characteristics of the injury. In addition, incident location and injured worker demographics do not have a significant effect on predicting claim severity. The presented model identifies higher injury risk groups and prevalent causes of incidents in work environments, allowing a more focused intervention effort in the food processing and feed milling sectors. In addition, it is applicable in forecasting cost of future claims and identifying factors that contribute to escalation of claims costs.
Available at: http://works.bepress.com/steven_freeman/70/
This proceeding is published as Kakhki, Fatemeh Davoudi, Steven A. Freeman, and Gretchen A. Mosher. "Classifying and Predicting Occupational Incident Severity." 2017 ATMAE Annual Conference, Cincinnati, OH, Nov. 1-3, 2017. Posted with permission.