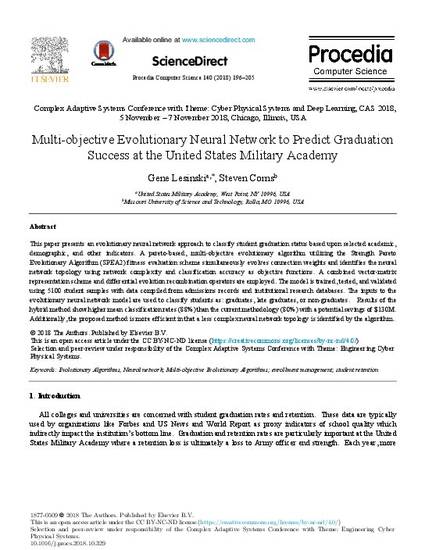
This paper presents an evolutionary neural network approach to classify student graduation status based upon selected academic, demographic, and other indicators. A pareto-based, multi-objective evolutionary algorithm utilizing the Strength Pareto Evolutionary Algorithm (SPEA2) fitness evaluation scheme simultaneously evolves connection weights and identifies the neural network topology using network complexity and classification accuracy as objective functions. A combined vector-matrix representation scheme and differential evolution recombination operators are employed. The model is trained, tested, and validated using 5100 student samples with data compiled from admissions records and institutional research databases. The inputs to the evolutionary neural network model are used to classify students as: graduates, late graduates, or non-graduates. Results of the hybrid method show higher mean classification rates (88%) than the current methodology (80%) with a potential savings of $130M. Additionally, the proposed method is more efficient in that a less complex neural network topology is identified by the algorithm.
- Enrollment management,
- Evolutionary Algorithms,
- Multi-objective Evolutionary Algorithms,
- Neural network,
- Student retention
Available at: http://works.bepress.com/steven-corns/24/