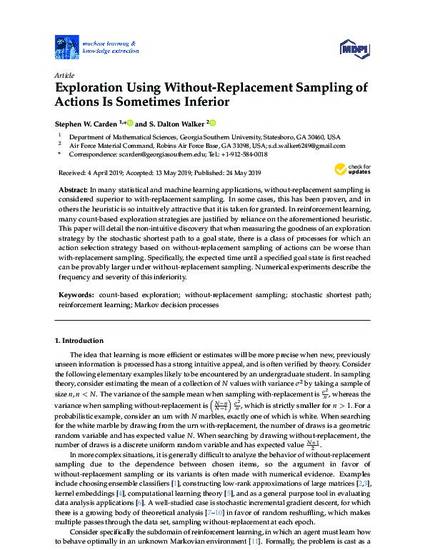
In many statistical and machine learning applications, without-replacement sampling is considered superior to with-replacement sampling. In some cases, this has been proven, and in others the heuristic is so intuitively attractive that it is taken for granted. In reinforcement learning, many count-based exploration strategies are justified by reliance on the aforementioned heuristic. This paper will detail the non-intuitive discovery that when measuring the goodness of an exploration strategy by the stochastic shortest path to a goal state, there is a class of processes for which an action selection strategy based on without-replacement sampling of actions can be worse than with-replacement sampling. Specifically, the expected time until a specified goal state is first reached can be provably larger under without-replacement sampling. Numerical experiments describe the frequency and severity of this inferiority.
Available at: http://works.bepress.com/stephen_carden/25/
© 2019 by the authors. Licensee MDPI, Basel, Switzerland. This article is an open access article distributed under the terms and conditions of the Creative Commons Attribution (CC BY) license (http://creativecommons.org/licenses/by/4.0/).