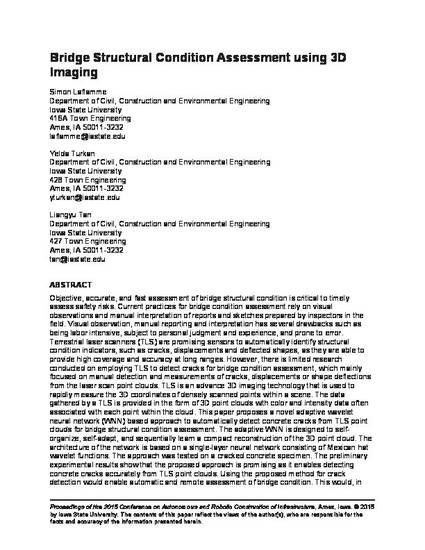
Objective, accurate, and fast assessment of bridge structural condition is critical to timely assess safety risks. Current practices for bridge condition assessment rely on visual observations and manual interpretation of reports and sketches prepared by inspectors in the field. Visual observation, manual reporting and interpretation has several drawbacks such as being labor intensive, subject to personal judgment and experience, and prone to error. Terrestrial laser scanners (TLS) are promising sensors to automatically identify structural condition indicators, such as cracks, displacements and deflected shapes, as they are able to provide high coverage and accuracy at long ranges. However, there is limited research conducted on employing TLS to detect cracks for bridge condition assessment, which mainly focused on manual detection and measurements of cracks, displacements or shape deflections from the laser scan point clouds. TLS is an advance 3D imaging technology that is used to rapidly measure the 3D coordinates of densely scanned points within a scene. The data gathered by a TLS is provided in the form of 3D point clouds with color and intensity data often associated with each point within the cloud. This paper proposes a novel adaptive wavelet neural network (WNN) based approach to automatically detect concrete cracks from TLS point clouds for bridge structural condition assessment. The adaptive WNN is designed to selforganize, self-adapt, and sequentially learn a compact reconstruction of the 3D point cloud. The architecture of the network is based on a single-layer neural network consisting of Mexican hat wavelet functions. The approach was tested on a cracked concrete specimen. The preliminary experimental results show that the proposed approach is promising as it enables detecting concrete cracks accurately from TLS point clouds. Using the proposed method for crack detection would enable automatic and remote assessment of bridge condition. This would, in turn, result in reducing costs associated with infrastructure management, and improving the overall quality of our infrastructure by enhancing maintenance operations.
Available at: http://works.bepress.com/simon_laflamme/45/
This is a paper from Proceedings of the 2015 Conference on Autonomous and Robotic Construction of Infrastructure, which can be found in full at: http://lib.dr.iastate.edu/intrans_reports/141/.