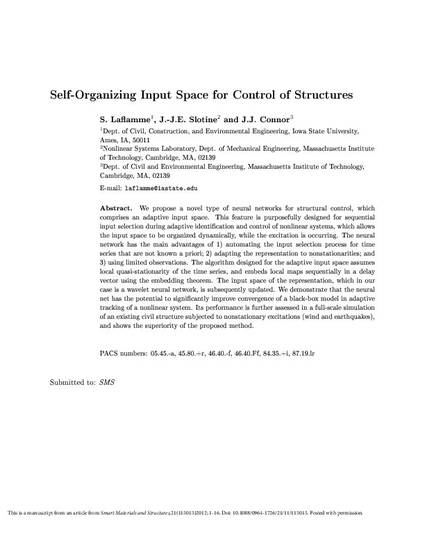
We propose a novel type of neural networks for structural control, which comprises an adaptive input space. This feature is purposefully designed for sequential input selection during adaptive identification and control of nonlinear systems, which allows the input space to be organized dynamically, while the excitation is occurring. The neural network has the main advantages of (1) automating the input selection process for time series that are not known a priori; (2) adapting the representation to nonstationarities; and (3) using limited observations. The algorithm designed for the adaptive input space assumes local quasi-stationarity of the time series, and embeds local maps sequentially in a delay vector using the embedding theorem. The input space of the representation, which in our case is a wavelet neural network, is subsequently updated. We demonstrate that the neural net has the potential to significantly improve convergence of a black-box model in adaptive tracking of a nonlinear system. Its performance is further assessed in a full-scale simulation of an existing civil structure subjected to nonstationary excitations (wind and earthquakes), and shows the superiority of the proposed method.
Available at: http://works.bepress.com/simon_laflamme/30/
This is a manuscript from an article from Smart Materials and Structures,21(115015)2012; 1-16. Doi: 10.1088/0964-1726/21/11/115015. Posted with permission.