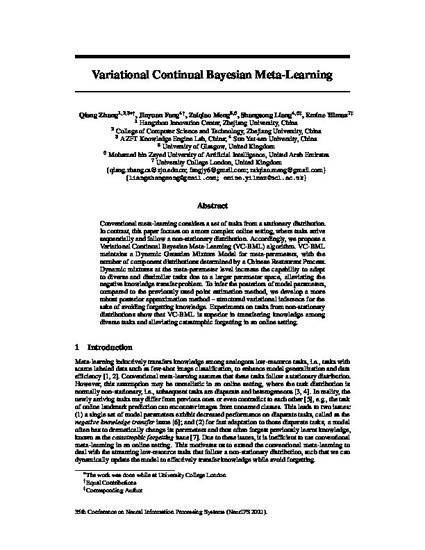
Conventional meta-learning considers a set of tasks from a stationary distribution. In contrast, this paper focuses on a more complex online setting, where tasks arrive sequentially and follow a non-stationary distribution. Accordingly, we propose a Variational Continual Bayesian Meta-Learning (VC-BML) algorithm. VC-BML maintains a Dynamic Gaussian Mixture Model for meta-parameters, with the number of component distributions determined by a Chinese Restaurant Process. Dynamic mixtures at the meta-parameter level increase the capability to adapt to diverse and dissimilar tasks due to a larger parameter space, alleviating the negative knowledge transfer problem. To infer the posteriors of model parameters, compared to the previously used point estimation method, we develop a more robust posterior approximation method – structured variational inference for the sake of avoiding forgetting knowledge. Experiments on tasks from non-stationary distributions show that VC-BML is superior in transferring knowledge among diverse tasks and alleviating catastrophic forgetting in an online setting. © 2021 Neural information processing systems foundation. All rights reserved.
- Gaussian distribution,
- Bayesian,
- Component distributions,
- Dynamic gaussian mixture models,
- Metalearning,
- Metaparameters,
- Nonstationary,
- Number of components,
- On-line setting,
- Parameter levels,
- Stationary distribution,
- Knowledge management
IR Deposit conditions: non-described