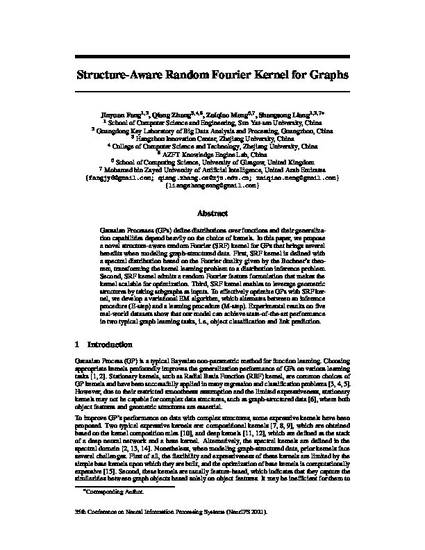
Gaussian Processes (GPs) define distributions over functions and their generalization capabilities depend heavily on the choice of kernels. In this paper, we propose a novel structure-aware random Fourier (SRF) kernel for GPs that brings several benefits when modeling graph-structured data. First, SRF kernel is defined with a spectral distribution based on the Fourier duality given by the Bochner's theorem, transforming the kernel learning problem to a distribution inference problem. Second, SRF kernel admits a random Fourier feature formulation that makes the kernel scalable for optimization. Third, SRF kernel enables to leverage geometric structures by taking subgraphs as inputs. To effectively optimize GPs with SRF kernel, we develop a variational EM algorithm, which alternates between an inference procedure (E-step) and a learning procedure (M-step). Experimental results on five real-world datasets show that our model can achieve state-of-the-art performance in two typical graph learning tasks, i.e., object classification and link prediction. © 2021 Neural information processing systems foundation. All rights reserved.
- Fourier transforms,
- Inference engines,
- Learning systems,
- Fourier,
- Gaussian Processes,
- Generalization capability,
- Graph structured data,
- Inference problem,
- Kernel learning,
- Learning problem,
- Novel structures,
- Spectral distribution,
- Structure-aware,
- Classification (of information)
IR Deposit conditions: non-described