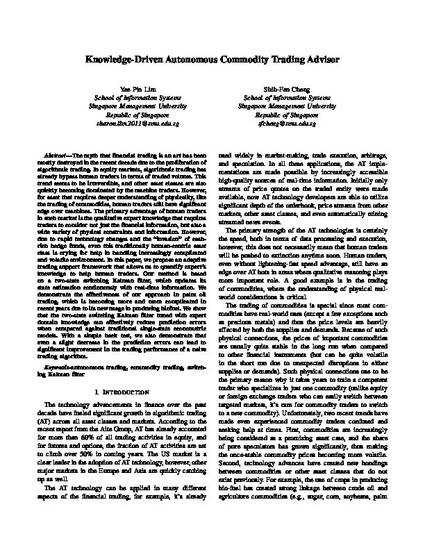
The myth that financial trading is an art has been mostly destroyed in the recent decade due to the proliferation of algorithmic trading. In equity markets, algorithmic trading has already bypass human traders in terms of traded volume. This trend seems to be irreversible, and other asset classes are also quickly becoming dominated by the machine traders. However, for asset that requires deeper understanding of physicality, like the trading of commodities, human traders still have significant edge over machines. The primary advantage of human traders in such market is the qualitative expert knowledge that requires traders to consider not just the financial information, but also a wide variety of physical constraints and information. However, due to rapid technology changes and the “invasion” of cashrich hedge funds, even this traditionally human-centric asset class is crying for help in handling increasingly complicated and volatile environment. In this paper, we propose an adaptive trading support framework that allows us to quantify expert’s knowledge to help human traders. Our method is based on a two-state switching Kalman filter, which updates its state estimation continuously with real-time information. We demonstrate the effectiveness of our approach in palm oil trading, which is becoming more and more complicated in recent years due to its new usage in producing biofuel.We show that the two-state switching Kalman filter tuned with expert domain knowledge can effectively reduce prediction errors when compared against traditional single-state econometric models. With a simple back test, we also demonstrate that even a slight decrease in the prediction errors can lead to significant improvement in the trading performance of a naive trading algorithm.
- autonomous trading,
- commodity trading,
- switching Kalman filter
Available at: http://works.bepress.com/sfcheng/25/