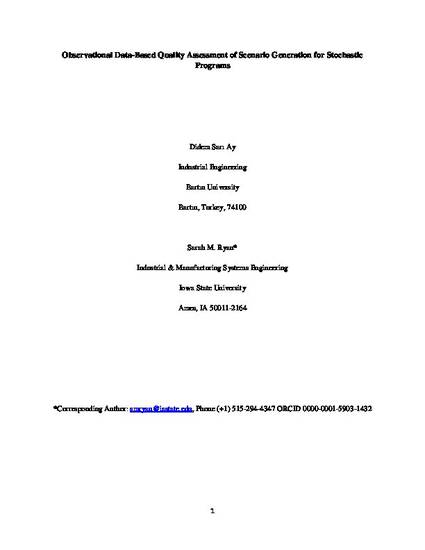
Article
Observational Data-Based Quality Assessment of Scenario Generation for Stochastic Programs
Computational Management Science
(2019)
Abstract
In minimization problems with uncertain parameters, cost savings can be achieved by solving stochastic programming (SP) formulations instead of using expected parameter values in a deterministic formulation. To obtain such savings, it is crucial to employ scenarios of high quality. An appealing way to assess the quality of scenarios produced by a given method is to conduct a re-enactment of historical instances in which the scenarios produced are used when solving the SP problem and the costs are assessed under the observed values of the uncertain parameters. Such studies are computationally very demanding. We propose two approaches for assessment of scenario generation methods using past instances that do not require solving SP instances. Instead of comparing scenarios to observations directly, these approaches consider the impact of each scenario in the SP problem. The methods are tested in simulation studies of server location and unit commitment, and then demonstrated in a case study of unit commitment with uncertain variable renewable energy generation.
Keywords
- Stochastic programming,
- Scenario generation method assessment,
- Scenario quality
Publication Date
2019
DOI
10.1007/s10287-019-00349-1
Citation Information
Didem Sari Ay and Sarah M. Ryan. "Observational Data-Based Quality Assessment of Scenario Generation for Stochastic Programs" Computational Management Science Vol. 16 (2019) p. 521 - 540 Available at: http://works.bepress.com/sarah_m_ryan/94/