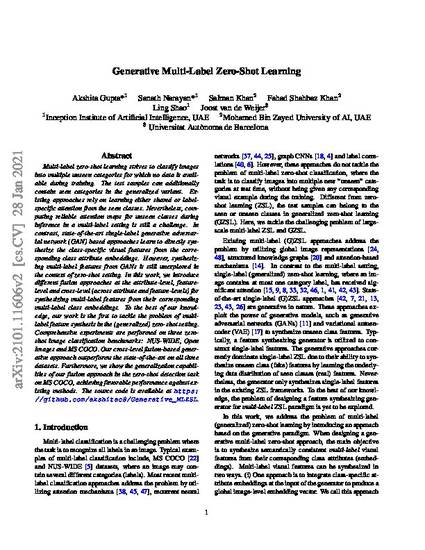
Multi-label zero-shot learning strives to classify images into multiple unseen categories for which no data is available during training. The test samples can additionally contain seen categories in the generalized variant. Existing approaches rely on learning either shared or label-specific attention from the seen classes. Nevertheless, computing reliable attention maps for unseen classes during inference in a multi-label setting is still a challenge. In contrast, state-of-the-art single-label generative adversarial network (GAN) based approaches learn to directly synthesize the class-specific visual features from the corresponding class attribute embeddings. However, synthesizing multi-label features from GANs is still unexplored in the context of zero-shot setting. In this work, we introduce different fusion approaches at the attribute-level, feature-level and cross-level (across attribute and feature-levels) for synthesizing multi-label features from their corresponding multi-label class embeddings. To the best of our knowledge, our work is the first to tackle the problem of multi-label feature synthesis in the (generalized) zero-shot setting. Comprehensive experiments are performed on three zero-shot image classification benchmarks: NUS-WIDE, Open Images and MS COCO. Our cross-level fusion-based generative approach outperforms the state-of-the-art on all three datasets. Furthermore, we show the generalization capabilities of our fusion approach in the zero-shot detection task on MS COCO, achieving favorable performance against existing methods. © 2021, CC BY-NC-SA.
- Computer Vision and Pattern Recognition (cs.CV)
Preprint: arXiv
Archived with thanks to arXiv
Preprint License: CC BY-NC-SA 4.0
Uploaded 25 March 2022