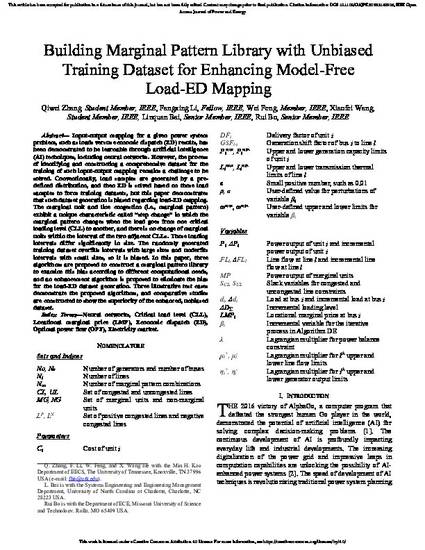
Input-output mapping for a given power system problem, such as loads versus economic dispatch (ED) results, has been demonstrated to be learnable through artificial intelligence (AI) techniques, including neural networks. However, the process of identifying and constructing a comprehensive dataset for the training of such input-output mapping remains a challenge to be solved. Conventionally, load samples are generated by a pre-defined distribution, and then ED is solved based on those load samples to form training datasets, but this paper demonstrates that such dataset generation is biased regarding load-ED mapping. The marginal unit and line congestion (i.e., marginal pattern) exhibit a unique characteristic called "step change" in which the marginal pattern changes when the load goes from one critical loading level (CLL) to another, and there is no change of marginal units within the interval of the two adjacent CLLs. Those loading intervals differ significantly in size. The randomly generated training dataset overfills intervals with large sizes and underfits intervals with small sizes, so it is biased. In this paper, three algorithms are proposed to construct a marginal pattern library to examine this bias according to different computational needs, and an enhancement algorithm is proposed to eliminate the bias for the load-ED dataset generation. Three illustrative test cases demonstrate the proposed algorithms, and comparative studies are constructed to show the superiority of the enhanced, unbiased dataset.
- Artificial Intelligence,
- Critical Load Level (CLL),
- Economic Dispatch (ED),
- Electricity Market,
- Libraries,
- Load Modeling,
- Loading,
- Locational Marginal Price (LMP),
- Neural Networks,
- Neural Networks,
- Optimal Power Flow (OPF),
- Prediction Algorithms,
- Training
Available at: http://works.bepress.com/rui-bo/110/