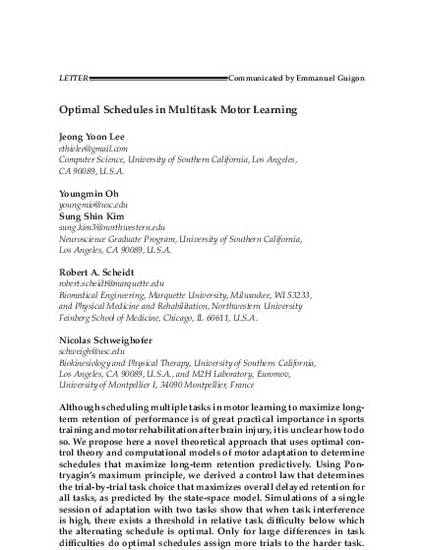
Although scheduling multiple tasks in motor learning to maximize long-term retention of performance is of great practical importance in sports training and motor rehabilitation after brain injury, it is unclear how to do so. We propose here a novel theoretical approach that uses optimal control theory and computational models of motor adaptation to determine schedules that maximize long-term retention predictively. Using Pontryagin’s maximum principle, we derived a control law that determines the trial-by-trial task choice that maximizes overall delayed retention for all tasks, as predicted by the state-space model. Simulations of a single session of adaptation with two tasks show that when task interference is high, there exists a threshold in relative task difficulty below which the alternating schedule is optimal. Only for large differences in task difficulties do optimal schedules assign more trials to the harder task. However, over the parameter range tested, alternating schedules yield long-term retention performance that is only slightly inferior to performance given by the true optimal schedules. Our results thus predict that in a large number of learning situations wherein tasks interfere, intermixing tasks with an equal number of trials is an effective strategy in enhancing long-term retention.
Available at: http://works.bepress.com/robert_scheidt/54/
Published version. Neural Computation, Vol. 28, No. 4 (April 2016): 667-685. DOI. © 2016 Massachusetts Institute of Technology. Used with permission.