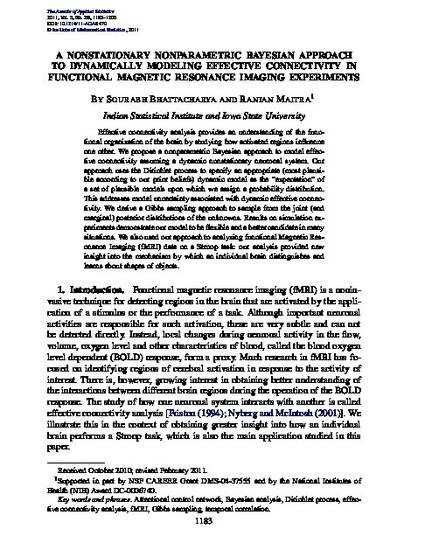
Effective connectivity analysis provides an understanding of the functional organization of the brain by studying how activated regions influence one other. We propose a nonparametric Bayesian approach to model effective connectivity assuming a dynamic nonstationary neuronal system. Our approach uses the Dirichlet process to specify an appropriate (most plausible according to our prior beliefs) dynamic model as the “expectation” of a set of plausible models upon which we assign a probability distribution. This addresses model uncertainty associated with dynamic effective connectivity. We derive a Gibbs sampling approach to sample from the joint (and marginal) posterior distributions of the unknowns. Results on simulation experiments demonstrate our model to be flexible and a better candidate in many situations. We also used our approach to analyzing functional Magnetic Resonance Imaging (fMRI) data on a Stroop task: our analysis provided new insight into the mechanism by which an individual brain distinguishes and learns about shapes of objects.
Available at: http://works.bepress.com/ranjan-maitra/1/
This is an article from The Annals of Applied Statistics 5 (2011): 1183, doi: 10.1214/11-AOAS470. Posted with permission.