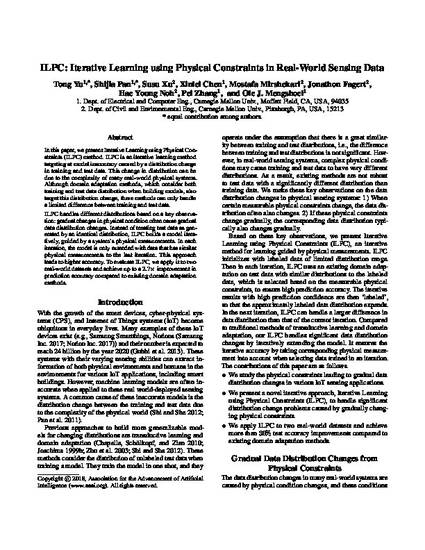
Article
ILPC: Iterative Learning using Physical Constraints in Real-World Sensing Data
AAAI Conference on Artificial Intelligence
(2018)
Abstract
In this paper, we present Iterative Learning using Physical Constraints (ILPC) method. ILPC is an iterative learning method targeting at model inaccuracy caused by a distribution change in training and test data. This change in distribution can be due to the complexity of many real-world physical systems. Although domain adaptation methods, which consider both training and test data distribution when building models, also target this distribution change, these methods can only handle a limited difference between training and test data. ILPC handles different distributions based on a key observation: gradual changes in physical condition often cause gradual data distribution changes. Instead of treating test data as generated by an identical distribution, ILPC builds a model iteratively, guided by a system’s physical measurements. In each iteration, the model is only extended with data that has similar physical measurements to the last iteration. This approach
leads to higher accuracy. To evaluate ILPC, we apply it to two real-world datasets and achieve up to a 2.7x improvement in prediction accuracy compared to existing domain adaptation methods.
Keywords
- Machine Learning,
- Distribution Change,
- Semi-Supervised Learning,
- Cyber-Physical Systems
Disciplines
Publication Date
February 3, 2018
Citation Information
Tong Yu, Shijia Pan, Susu Xu, Xinlei Chen, et al.. "ILPC: Iterative Learning using Physical Constraints in Real-World Sensing Data" AAAI Conference on Artificial Intelligence (2018) Available at: http://works.bepress.com/ole_mengshoel/75/