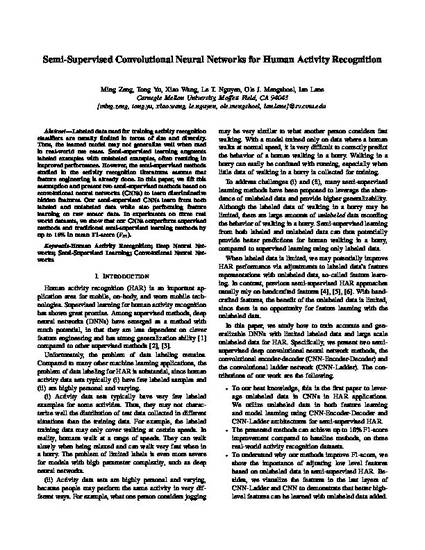
Article
Semi-Supervised Convolutional Neural Networks for Human Activity Recognition
IEEE BigData 2017
(2017)
Abstract
Labeled data used for training activity recognition classifiers are usually limited in terms of size and diversity. Thus, the learned model may not generalize well when used in real-world use cases. Semi-supervised learning augments labeled examples with unlabeled examples, often resulting in improved performance. However, the semi-supervised methods studied in the activity recognition literature assume that feature engineering is already done. In this paper, we lift this assumption and present two semi-supervised methods based on convolutional neural networks (CNNs) to learn discriminative hidden features. Our semi-supervised CNNs learn from both
labeled and unlabeled data while also performing feature learning on raw sensor data. In experiments on three real world datasets, we show that our CNNs outperform supervised methods and traditional semi-supervised learning methods by up to 18% in mean F1-score
Keywords
- Human Activity Recognition,
- Deep Learning,
- Convolutional Neural Network,
- Semi-Supervised Learning,
- Machine Learning,
- Human-Computer Interaction
Disciplines
Publication Date
December, 2017
Citation Information
Ming Zeng, Tong Yu, Xiao Wang, Le T. Nguyen, et al.. "Semi-Supervised Convolutional Neural Networks for Human Activity Recognition" IEEE BigData 2017 (2017) p. 522 - 529 Available at: http://works.bepress.com/ole_mengshoel/73/