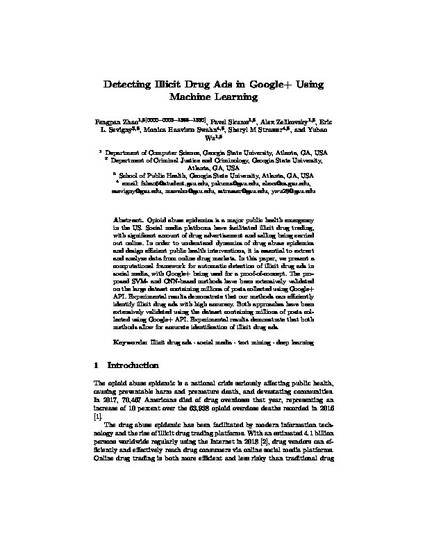
Opioid abuse epidemics is a major public health emergency in the US. Social media platforms have facilitated illicit drug trading, with significant amount of drug advertisement and selling being carried out online. In order to understand dynamics of drug abuse epidemics and design efficient public health interventions, it is essential to extract and analyze data from online drug markets. In this paper, we present a computational framework for automatic detection of illicit drug ads in social media, with Google+ being used for a proof-of-concept. The proposed SVM- and CNN-based methods have been extensively validated on the large dataset containing millions of posts collected using Google+ API. Experimental results demonstrate that our methods can efficiently identify illicit drug ads with high accuracy. Both approaches have been extensively validated using the dataset containing millions of posts collected using Google+ API. Experimental results demonstrate that both methods allow for accurate identification of illicit drug ads.
Publisher version available at https://doi.org/10.1007/978-3-030-20242-2_15. The archived and publisher version may slightly differ due to copyediting.