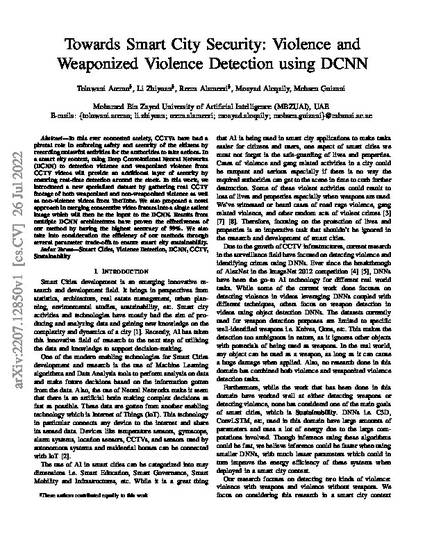
In this ever connected society, CCTVs have had a pivotal role in enforcing safety and security of the citizens by recording unlawful activities for the authorities to take actions. In a smart city context, using Deep Convolutional Neural Networks (DCNN) to detection violence and weaponized violence from CCTV videos will provide an additional layer of security by ensuring real-time detection around the clock. In this work, we introduced a new specialised dataset by gathering real CCTV footage of both weaponized and non-weaponized violence as well as non-violence videos from YouTube. We also proposed a novel approach in merging consecutive video frames into a single salient image which will then be the input to the DCNN. Results from multiple DCNN architectures have proven the effectiveness of our method by having the highest accuracy of 99%. We also take into consideration the efficiency of our methods through several parameter trade-offs to ensure smart city sustainability. © 2022, CC BY.
- CCTV,
- DCNN,
- Smart Cities,
- Sustainability,
- Violence Detection
Preprint: arXiv
Archived with thanks to arXiv
Preprint License: CC by 4.0
Uploaded 24 August 2022