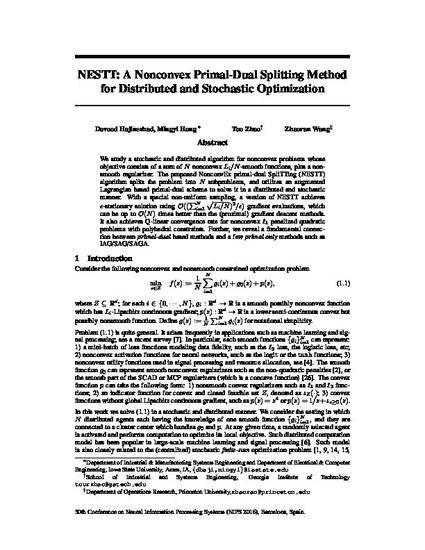
We study a stochastic and distributed algorithm for nonconvex problems whose objective consists a sum N/ nonconvex Li/N/ smooth functions, plus a nonsmooth regularizer. The proposed NonconvEx primal-dual SpliTTing (NESTT) algorithm splits the problem into N/ subproblems, and utilizes an augmented Lagrangian based primal-dual scheme to solve it in a distributed and stochastic manner. With a special non-uniform sampling, a version of NESTT achieves e-1 stationary solution using...gradient evaluations, which can be up to O(N)/ times better than the (proximal) gradient descent methods. It also achieves Q-linear convergence rate for nonconvex l1 penalized quadratic problems with polyhedral constraints. Further, we reveal a fundamental connection between {\it primal-dual} based methods and a few {\it primal only} methods such as IAG/SAG/SAGA.
Available at: http://works.bepress.com/mingyi_hong/29/
This is a proceeding from the 30th Conference on Neural Information Processing Systems (2016). Posted with permission.