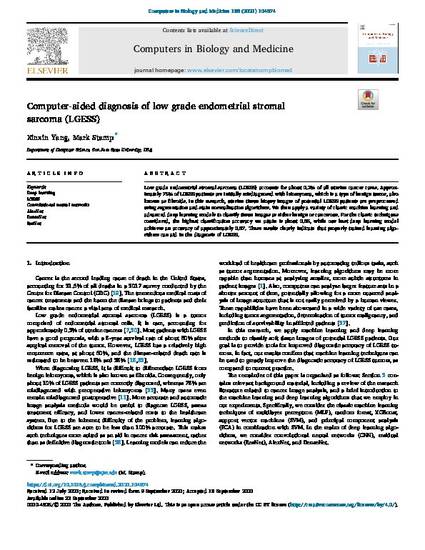
Low grade endometrial stromal sarcoma (LGESS) accounts for about 0.2% of all uterine cancer cases. Approximately 75% of LGESS patients are initially misdiagnosed with leiomyoma, which is a type of benign tumor, also known as fibroids. In this research, uterine tissue biopsy images of potential LGESS patients are preprocessed using segmentation and stain normalization algorithms. We then apply a variety of classic machine learning and advanced deep learning models to classify tissue images as either benign or cancerous. For the classic techniques considered, the highest classification accuracy we attain is about 0.85, while our best deep learning model achieves an accuracy of approximately 0.87. These results clearly indicate that properly trained learning algorithms can aid in the diagnosis of LGESS.
- Deep learning,
- LGESS,
- Convolutional neural networks,
- AlexNet,
- DenseNet,
- ResNet
Available at: http://works.bepress.com/mark_stamp/118/
This is the Version of Record and can also be read online here.