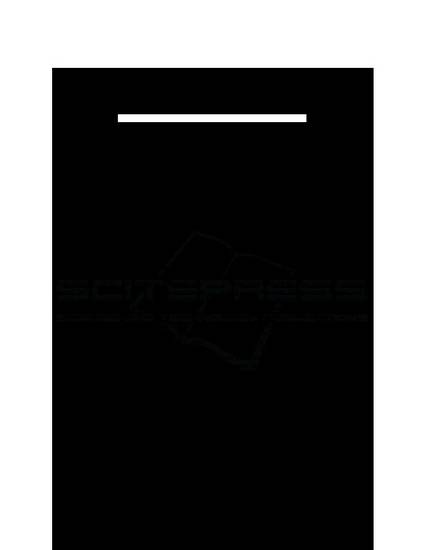
Contribution to Book
On the Effectiveness of Generic Malware Models
Proceedings of the 15th International Joint Conference on e-Business and Telecommunications (ICETE 2018) - Volume 1: DCNET, ICE-B, OPTICS, SIGMAP and WINSYS
Document Type
Conference Proceeding
Publication Date
1-1-2018
Editor
Christian Callegari, Marten van Sinderen, Paulo Novais, Panagiotis Sarigiannidis, Sebastiano Battiato, Ángel Serrano Sánchez de León, Pascal Lorenz, and Mohammad S. Obaidat
Disciplines
Abstract
Malware detection based on machine learning typically involves training and testing models for each malware family under consideration. While such an approach can generally achieve good accuracy, it requires many classification steps, resulting in a slow, inefficient, and potentially impractical process. In contrast, classifying samples as malware or benign based on more generic “families” would be far more efficient. However, extracting common features from extremely general malware families will likely result in a model that is too generic to be useful. In this research, we perform controlled experiments to determine the tradeoff between generality and accuracy—over a variety of machine learning techniques—based on n-gram features.
Creative Commons License
Creative Commons Attribution-Noncommercial-No Derivative Works 4.0
Citation Information
Naman Bagga, Fabio Di Troia and Mark Stamp. "On the Effectiveness of Generic Malware Models" Proceedings of the 15th International Joint Conference on e-Business and Telecommunications (ICETE 2018) - Volume 1: DCNET, ICE-B, OPTICS, SIGMAP and WINSYS (2018) p. 442 - 450 Available at: http://works.bepress.com/mark_stamp/117/
This paper can also be read online here.