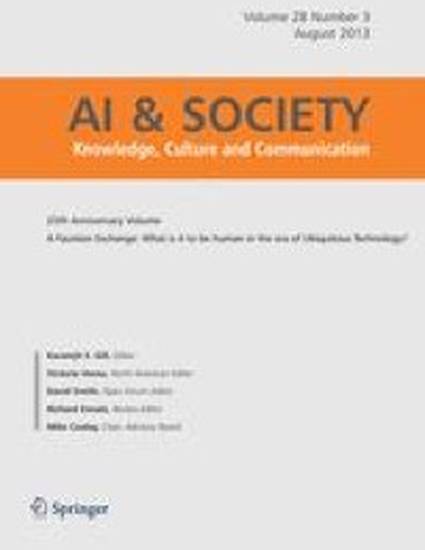
Article
Detecting racial inequalities in criminal justice: towards an equitable deep learning approach for generating and interpreting racial categories using mugshots
AI & SOCIETY
(2022)
Abstract
Recent events have highlighted large-scale systemic racial disparities in U.S. criminal justice based on race and other demographic characteristics. Although criminological datasets are used to study and document the extent of such disparities, they often lack key information, including arrestees’ racial identification. As AI technologies are increasingly used by criminal justice agencies to make predictions about outcomes in bail, policing, and other decision-making, a growing literature suggests that the current implementation of these systems may perpetuate racial inequalities. In this paper, we argue that AI technologies should be investigated to understand how they recognize racial categories and whether they can be harnessed to fill missing race data. By bridging this gap, we can work towards better understanding racial inequalities in a wide range of contexts, most notably criminal justice. Using a multidisciplinary perspective, we rethink the design and methodology used in facial processing technology (FPT) based on supervised deep learning model (DLM) image classification. By modifying standard FPT pipelines to tackle multiple sources of DLM bias, we propose an experimental methodology based on ethical AI principles to generate binary (Black and White) racial categories using mugshots. We go beyond simply reporting DLM accuracies and address fundamental issues such as generalizability and interpretability by using a “self-auditing” approach. First, we evaluate the inference performances of 42 fine-tuned DLMs using unseen test images from the same dataset but subject to varying data augmentations. Next, to interpret and validate our methodological approach, we apply gradient-based saliency maps to assess the consistency of facial region relevance and attribution. Finally, drawing upon insights from three areas (computer science, sociology, and law), we investigate the efficacy of our DLM-based method as a tool for detecting racial inequalities in criminal justice.
Disciplines
Publication Date
2022
DOI
10.1007/S00146-022-01440-Z
Citation Information
Rahul Kumar Dass, Nick Petersen, Marisa Omori, Tamara Rice Lave, et al.. "Detecting racial inequalities in criminal justice: towards an equitable deep learning approach for generating and interpreting racial categories using mugshots" AI & SOCIETY (2022) Available at: http://works.bepress.com/marisa-omori/31/