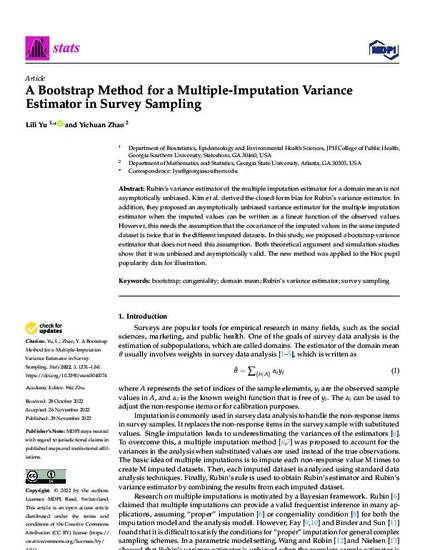
Rubin’s variance estimator of the multiple imputation estimator for a domain mean is not asymptotically unbiased. Kim et al. derived the closed-form bias for Rubin’s variance estimator. In addition, they proposed an asymptotically unbiased variance estimator for the multiple imputation estimator when the imputed values can be written as a linear function of the observed values. However, this needs the assumption that the covariance of the imputed values in the same imputed dataset is twice that in the different imputed datasets. In this study, we proposed a bootstrap variance estimator that does not need this assumption. Both theoretical argument and simulation studies show that it was unbiased and asymptotically valid. The new method was applied to the Hox pupil popularity data for illustration.
Copyright: © 2022 by the authors. Licensee MDPI, Basel, Switzerland. This article is an open access article distributed under the terms and conditions of the Creative Commons Attribution (CC BY) license (https:// creativecommons.org/licenses/by/ 4.0/).
Available at: http://works.bepress.com/lili-yu/90/
Georgia Southern University faculty member, Lili Yu co-authored A Bootstrap Method for a Multiple-Imputation Variance Estimator in Survey Sampling.