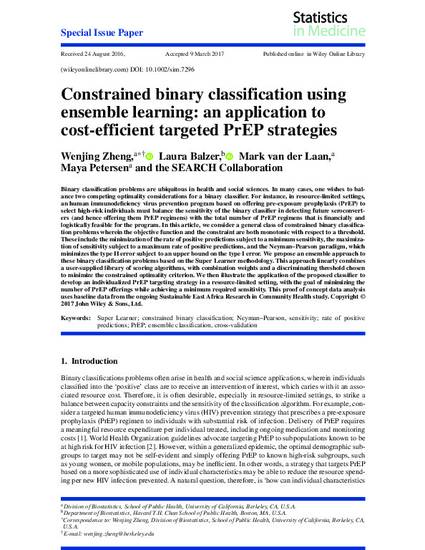
Article
Constrained binary classification using ensemble learning: an application to cost-efficient targeted PrEP strategies
Statistics in Medicine
(2017)
Abstract
Binary classification problems are ubiquitous in health and social sciences. In many cases, one wishes to balance two competing optimality considerations for a binary classifier. For instance, in resource-limited settings, an human immunodeficiency virus prevention program based on offering pre-exposure prophylaxis (PrEP) to select high-risk individuals must balance the sensitivity of the binary classifier in detecting future seroconverters (and hence offering them PrEP regimens) with the total number of PrEP regimens that is financially and logistically feasible for the program. In this article, we consider a general class of constrained binary classification problems wherein the objective function and the constraint are both monotonic with respect to a threshold. These include the minimization of the rate of positive predictions subject to a minimum sensitivity, the maximization of sensitivity subject to a maximum rate of positive predictions, and the Neyman–Pearson paradigm, which minimizes the type II error subject to an upper bound on the type I error. We propose an ensemble approach to these binary classification problems based on the Super Learner methodology. This approach linearly combines a user-supplied library of scoring algorithms, with combination weights and a discriminating threshold chosen to minimize the constrained optimality criterion. We then illustrate the application of the proposed classifier to develop an individualized PrEP targeting strategy in a resource-limited setting, with the goal of minimizing the number of PrEP offerings while achieving a minimum required sensitivity. This proof of concept data analysis uses baseline data from the ongoing Sustainable East Africa Research in Community Health study.
Disciplines
Publication Date
March, 2017
Citation Information
Wenjing Zheng, Laura Balzer, Mark J. van der Laan, Maya Petersen, et al.. "Constrained binary classification using ensemble learning: an application to cost-efficient targeted PrEP strategies" Statistics in Medicine (2017) Available at: http://works.bepress.com/laura_balzer/46/