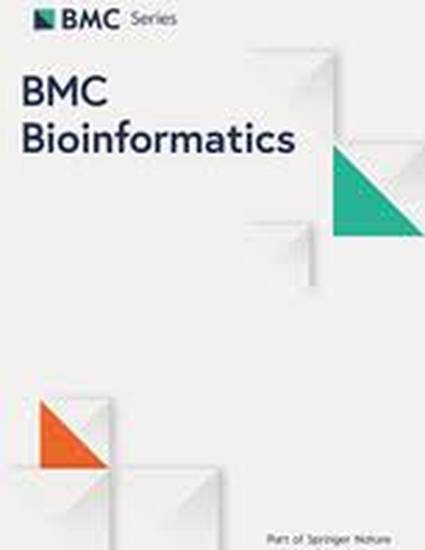
Microarray technology is increasingly used to identify potential biomarkers for cancer prognostics and diagnostics. Previously, we have developed the iterative Bayesian Model Averaging (BMA) algorithm for use in classification. Here, we extend the iterative BMA algorithm for application to survival analysis on high-dimensional microarray data. The main goal in applying survival analysis to microarray data is to determine a highly predictive model of patients' time to event (such as death, relapse, or metastasis) using a small number of selected genes. Our multivariate procedure combines the effectiveness of multiple contending models by calculating the weighted average of their posterior probability distributions. Our results demonstrate that our iterative BMA algorithm for survival analysis achieves high prediction accuracy while consistently selecting a small and cost-effective number of predictor genes.
Available at: http://works.bepress.com/ky-yeung/27/