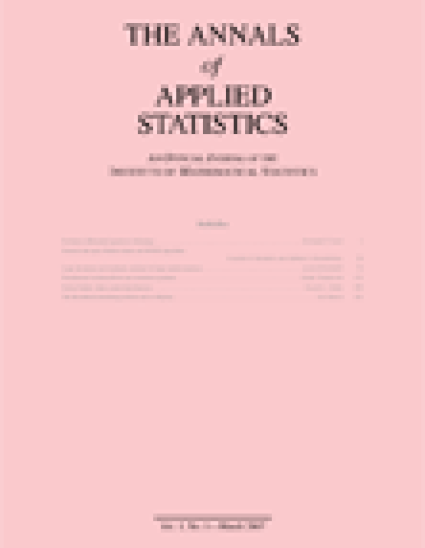
The NIH Library of Integrated Network-based Cellular Signatures (LINCS) contains gene expression data from over a million experiments, using Luminex Bead technology. Only 500 colors are used to measure the expression levels of the 1000 landmark genes measured, and the data for the resulting pairs of genes are deconvolved. The raw data are sometimes inadequate for reliable deconvolution, leading to artifacts in the final processed data. These include the expression levels of paired genes being flipped or given the same value and clusters of values that are not at the true expression level. We propose a new method called model-based clustering with data correction (MCDC) that is able to identify and correct these three kinds of artifacts simultaneously. We show that MCDC improves the resulting gene expression data in terms of agreement with external baselines, as well as improving results from subsequent analysis.
Available at: http://works.bepress.com/ky-yeung/14/