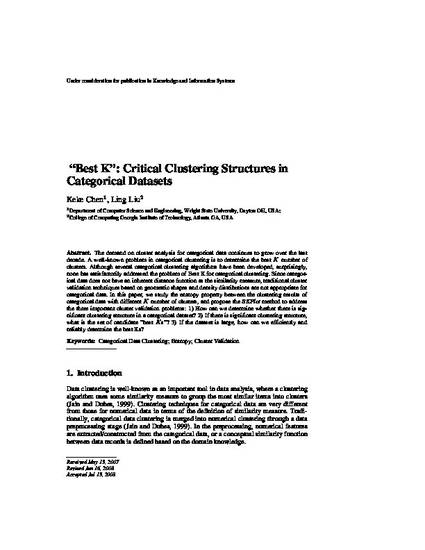
The demand on cluster analysis for categorical data continues to grow over the last decade. A well-known problem in categorical clustering is to determine the best K number of clusters. Although several categorical clustering algorithms have been developed, surprisingly, none has satisfactorily addressed the problem of best K for categorical clustering. Since categorical data does not have an inherent distance function as the similarity measure, traditional cluster validation techniques based on geometric shapes and density distributions are not appropriate for categorical data. In this paper, we study the entropy property between the clustering results of categorical data with different K number of clusters, and propose the BKPlot method to address the three important cluster validation problems: (1) How can we determine whether there is significant clustering structure in a categorical dataset? (2) If there is significant clustering structure, what is the set of candidate “best Ks”? (3) If the dataset is large, how can we efficiently and reliably determine the best Ks?
Available at: http://works.bepress.com/keke_chen/12/
The featured PDF document is the unpublished, peer-reviewed version of this article.
The featured abstract was published in the final version of this article, which appeared in Knowledge and Information Systems, volume 20, issue 1, pp. 1-33 and may be found at http://link.springer.com/article/10.1007%2Fs10115-008-0159-x.