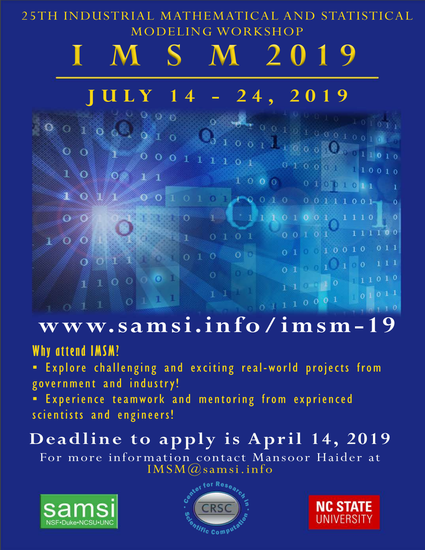
Presentation
Analysis and Visualization of Continuous Glucose Monitoring Data
2019 Industrial Math/Stat Modeling (IMSM) Workshop for Graduate Students
(2019)
Abstract
Continuous glucose monitors (CGMs) are increasingly used to assess and manage glucose levels in patients with diabetes. CGMs provide an alternative to the daily self-monitoring of blood glucose (SMBG) which proves to be painful, inconvenient, costly, and difficult to maintain. Instead, CGMs use less invasive, subcutaneous sensors to measure interstitial glucose levels at 5-minute intervals throughout the day and night. The collection of regularly timed, short interval data also leads to a better understanding of glucose patterns and trends. These data-rich glucose monitoring are percolating as primary or secondary outcomes into randomized clinical trials (RCTs) requiring a rethinking of ordinary statistical techniques.
CGMs generate very large and complex data sets (288 measurements per 24 hours). To analyze these data, researchers tend to derive summary variables that are then used in subsequent analyses, e.g. Area Under the Curve (AUC) or time spent above, below or within a specified target.[1,2] Although these indices are informative, they do remove much of the potential additional information embedded in this temporal data. This includes not only an indication of glucose levels at or across specific points in time but also measures of change (velocity), rate of change (acceleration), and variability. More advanced statistical techniques are required to access this additional information and making it available for clinical interpretation and application.
The goal of this project is to encourage the students to go beyond the usual summary statistics to determine individual and treatment group differences over time, and instead provide a comprehensive analysis and visualization framework using one or more novel statistical techniques that incorporate the temporal nature of CGM data (or an equivalent type data set in the event CGM data is not available for public use at the time of the workshop). One such technique could be Functional Data Analysis (FDA) [3] as it’s capable of summarizing temporal trends of continuously recorded measurements in a form that is amenable to subsequent multivariable statistical analysis [4].
Citations
[1] Danne et al 2017. International Consensus on Use of Continuous Glucose Monitoring. Diabetes Care, 40 (12): 1631-1640; DOI: 10.2337/dc17-1600
[2] Hirsch et al 2018. Role of Continuous Glucose Monitoring in Diabetes Treatment. American Diabetes Association https://professional.diabetes.org/sites/professional.diabetes.org/files/media/final_adaabbott_cgm_compendium_final.pdf
[3] Ramsay, James O., and Bernard W. Silverman. Applied functional data analysis: methods and case studies. Springer, 2007.
[4] CRAN Task View: Functional Data Analysis https://cran.r-project.org/web/views/FunctionalData.html
Disciplines
Publication Date
July, 2019
Location
Raleigh, NC
Citation Information
Agustin Calaroni, Petra LeBeau, Pulong Ma, Yin-Jen Chen, et al.. "Analysis and Visualization of Continuous Glucose Monitoring Data" 2019 Industrial Math/Stat Modeling (IMSM) Workshop for Graduate Students (2019) Available at: http://works.bepress.com/juna-goo/2/