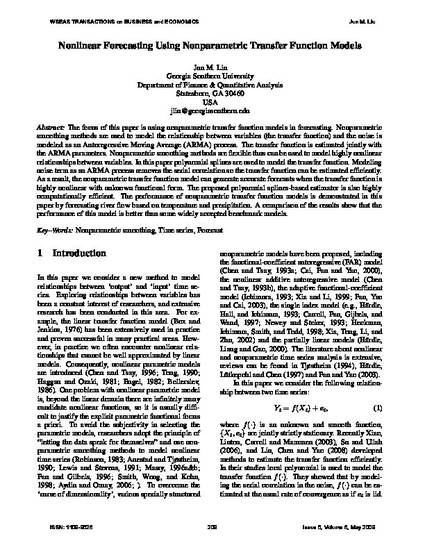
Article
Nonlinear Forecasting Using Nonparametric Transfer Function Models
WSEAS Transactions on Business and Economics
(2009)
Abstract
The focus of this paper is using nonparametric transfer function models in forecasting. Nonparametric smoothing methods are used to model the relationship between variables (the transfer function) and the noise is modeled as an Autoregressive Moving Average (ARMA) process. The transfer function is estimated jointly with the ARMA parameters. Nonparametric smoothing methods are flexible thus can be used to model highly nonlinear relationships between variables. In this paper polynomial splines are used to model the transfer function. Modeling noise term as an ARMA process removes the serial correlation so the transfer function can be estimated efficiently. As a result, the nonparametric transfer function model can generate accurate forecasts when the transfer function is highly nonlinear with unknown functional form. The proposed polynomial splines-based estimator is also highly computationally efficient. The performance of nonparametric transfer function models is demonstrated in this paper by forecasting river flow based on temperature and precipitation. A comparison of the results show that the performance of this model is better than some widely accepted benchmark models.
Keywords
- Nonparametric smoothing,
- Time series,
- Forecast
Disciplines
Publication Date
May, 2009
Publisher Statement
Copyright © 2017 - All Rights Reserved - WSEAS - World Scientific and Engineering Academy and Society
Citation Information
Jun Liu. "Nonlinear Forecasting Using Nonparametric Transfer Function Models" WSEAS Transactions on Business and Economics Vol. 6 Iss. 5 (2009) p. 209 - 2018 ISSN: 1109-9526 Available at: http://works.bepress.com/jun_liu/7/