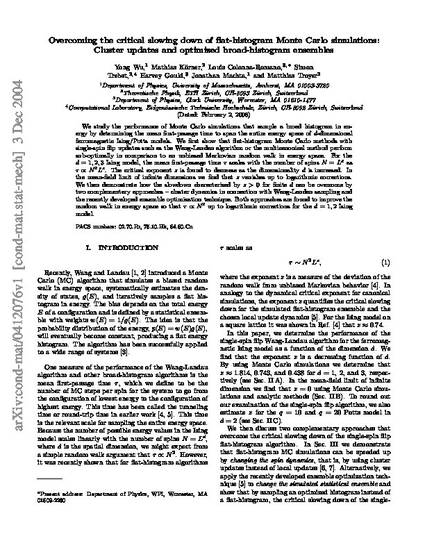
We study the performance of Monte Carlo simulations that sample a broad histogram in energy by determining the mean first-passage time to span the entire energy space of d-dimensional ferromagnetic Ising/Potts models. We first show that flat-histogram Monte Carlo methods with single-spin flip updates such as the Wang-Landau algorithm or the multicanonical method perform suboptimally in comparison to an unbiased Markovian random walk in energy space. For the d=1, 2, 3 Ising model, the mean first-passage time τ scales with the number of spins N=Ld as τ∝N2Lz. The exponent z is found to decrease as the dimensionality d is increased. In the mean-field limit of infinite dimensions we find that z vanishes up to logarithmic corrections. We then demonstrate how the slowdown characterized by z>0 for finite d can be overcome by two complementary approaches—cluster dynamics in connection with Wang-Landau sampling and the recently developed ensemble optimization technique. Both approaches are found to improve the random walk in energy space so that τ∝N2 up to logarithmic corrections for the d=1, 2 Ising model.
Available at: http://works.bepress.com/joonathan_machta/5/