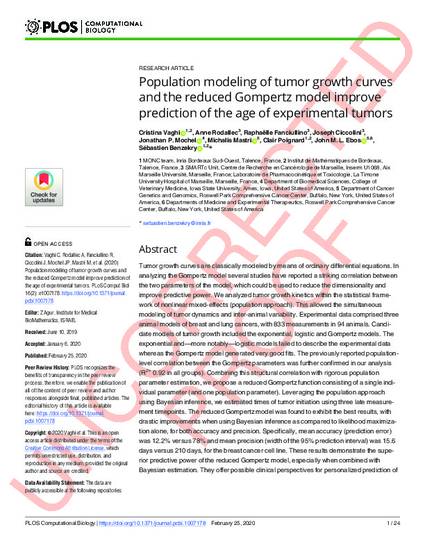
Tumor growth curves are classically modeled by means of ordinary differential equations. In analyzing the Gompertz model several studies have reported a striking correlation between the two parameters of the model, which could be used to reduce the dimensionality and improve predictive power. We analyzed tumor growth kinetics within the statistical framework of nonlinear mixed-effects (population approach). This allowed the simultaneous modeling of tumor dynamics and inter-animal variability. Experimental data comprised three animal models of breast and lung cancers, with 833 measurements in 94 animals. Candidate models of tumor growth included the exponential, logistic and Gompertz models. The exponential and—more notably—logistic models failed to describe the experimental data whereas the Gompertz model generated very good fits. The previously reported population-level correlation between the Gompertz parameters was further confirmed in our analysis (R2> 0.92 in all groups). Combining this structural correlation with rigorous population parameter estimation, we propose a reduced Gompertz function consisting of a single individual parameter (and one population parameter). Leveraging the population approach using Bayesian inference, we estimated times of tumor initiation using three late measurement timepoints. The reduced Gompertz model was found to exhibit the best results, with drastic improvements when using Bayesian inference as compared to likelihood maximization alone, for both accuracy and precision. Specifically, mean accuracy (prediction error) was 12.2% versus 78% and mean precision (width of the 95% prediction interval) was 15.6 days versus 210 days, for the breast cancer cell line. These results demonstrate the superior predictive power of the reduced Gompertz model, especially when combined with Bayesian estimation. They offer possible clinical perspectives for personalized prediction of the age of a tumor from limited data at diagnosis. The code and data used in our analysis are publicly available at https://github.com/cristinavaghi/plumky.
Available at: http://works.bepress.com/jonathan-mochel/68/
This is a manuscript of an article published as Vaghi, Cristina, Anne Rodallec, Raphaëlle Fanciullino, Joseph Ciccolini, Jonathan P. Mochel, Michalis Mastri, Clair Poignard, John ML Ebos, and Sébastien Benzekry. "Population modeling of tumor growth curves and the reduced Gompertz model improve prediction of the age of experimental tumors." PLOS Computational Biology 16, no. 2 (2020): e1007178. DOI: 10.1371/journal.pcbi.1007178. Posted with permission.