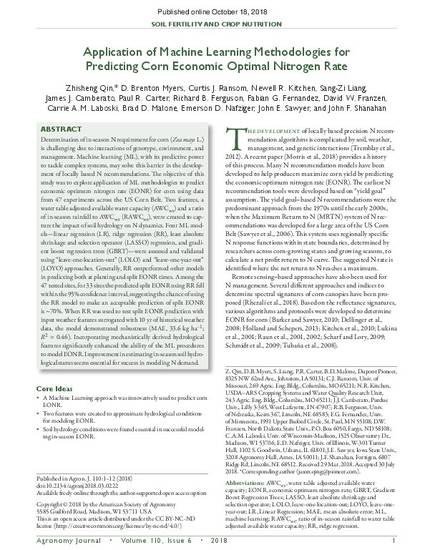
Determination of in-season N requirement for corn (Zea mays L.) is challenging due to interactions of genotype, environment, and management. Machine learning (ML), with its predictive power to tackle complex systems, may solve this barrier in the development of locally based N recommendations. The objective of this study was to explore application of ML methodologies to predict economic optimum nitrogen rate (EONR) for corn using data from 47 experiments across the US Corn Belt. Two features, a water table adjusted available water capacity (AWCwt) and a ratio of in-season rainfall to AWCwt (RAWCwt), were created to capture the impact of soil hydrology on N dynamics. Four ML models—linear regression (LR), ridge regression (RR), least absolute shrinkage and selection operator (LASSO) regression, and gradient boost regression trees (GBRT)—were assessed and validated using “leave-one-location-out” (LOLO) and “leave-one-year-out” (LOYO) approaches. Generally, RR outperformed other models in predicting both at planting and split EONR times. Among the 47 tested sites, for 33 sites the predicted split EONR using RR fell within the 95% confidence interval, suggesting the chance of using the RR model to make an acceptable prediction of split EONR is ∼70%. When RR was used to test split EONR prediction with input weather features surrogated with 10 yr of historical weather data, the model demonstrated robustness (MAE, 33.6 kg ha–1; R2 = 0.46). Incorporating mechanistically derived hydrological features significantly enhanced the ability of the ML procedures to model EONR. Improvement in estimating in-season soil hydrological status seems essential for success in modeling N demand.
Available at: http://works.bepress.com/john-sawyer/404/
This article is published as Li, Chao, Dermot J. Hayes, and Keri L. Jacobs. "Biomass for Bioenergy: Optimal Collection Mechanisms and Pricing when Feedstock Supply Does Not Equal Availability." Energy Economics 110 (2018): 1-12. doi: 10.2134/agronj2018.03.0222.