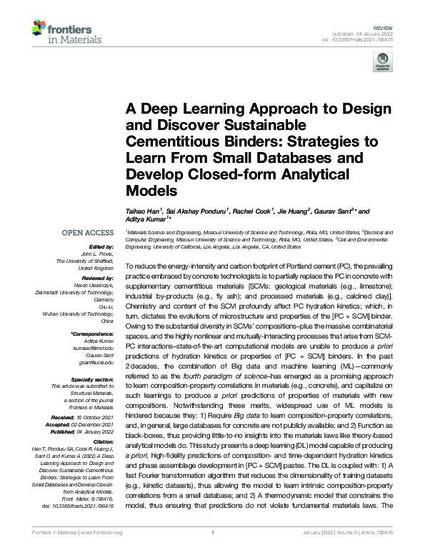
To reduce the energy-intensity and carbon footprint of Portland cement (PC), the prevailing practice embraced by concrete technologists is to partially replace the PC in concrete with supplementary cementitious materials [SCMs: geological materials (e.g., limestone); industrial by-products (e.g., fly ash); and processed materials (e.g., calcined clay)]. Chemistry and content of the SCM profoundly affect PC hydration kinetics; which, in turn, dictates the evolutions of microstructure and properties of the [PC + SCM] binder. Owing to the substantial diversity in SCMs' compositions–plus the massive combinatorial spaces, and the highly nonlinear and mutually-interacting processes that arise from SCM-PC interactions–state-of-the-art computational models are unable to produce a priori predictions of hydration kinetics or properties of [PC + SCM] binders. In the past 2 decades, the combination of Big data and machine learning (ML)—commonly referred to as the fourth paradigm of science–has emerged as a promising approach to learn composition-property correlations in materials (e.g., concrete), and capitalize on such learnings to produce a priori predictions of properties of materials with new compositions. Notwithstanding these merits, widespread use of ML models is hindered because they: 1) Require Big data to learn composition-property correlations, and, in general, large databases for concrete are not publicly available; and 2) Function as black-boxes, thus providing little-to-no insights into the materials laws like theory-based analytical models do. This study presents a deep learning (DL) model capable of producing a priori, high-fidelity predictions of composition- and time-dependent hydration kinetics and phase assemblage development in [PC + SCM] pastes. The DL is coupled with: 1) A fast Fourier transformation algorithm that reduces the dimensionality of training datasets (e.g., kinetic datasets), thus allowing the model to learn intrinsic composition-property correlations from a small database; and 2) A thermodynamic model that constrains the model, thus ensuring that predictions do not violate fundamental materials laws. The training and outcomes of the DL are ultimately leveraged to develop a simple, easy-to-use, closed-form analytical model capable of predicting hydration kinetics and phase assemblage development in [PC + SCM] pastes, using their initial composition and mixture design as inputs.
- deep learning,
- hydration kinetic,
- prediction,
- sustaianability,
- thermodynamics
Available at: http://works.bepress.com/jie-huang/211/
National Science Foundation, Grant 1661609