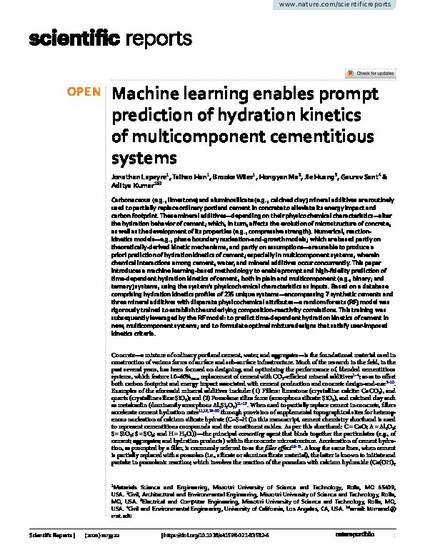
Carbonaceous (e.g., limestone) and aluminosilicate (e.g., calcined clay) mineral additives are routinely used to partially replace ordinary portland cement in concrete to alleviate its energy impact and carbon footprint. These mineral additives—depending on their physicochemical characteristics—alter the hydration behavior of cement; which, in turn, affects the evolution of microstructure of concrete, as well as the development of its properties (e.g., compressive strength). Numerical, reaction-kinetics models—e.g., phase boundary nucleation-and-growth models; which are based partly on theoretically-derived kinetic mechanisms, and partly on assumptions—are unable to produce a priori prediction of hydration kinetics of cement; especially in multicomponent systems, wherein chemical interactions among cement, water, and mineral additives occur concurrently. This paper introduces a machine learning-based methodology to enable prompt and high-fidelity prediction of time-dependent hydration kinetics of cement, both in plain and multicomponent (e.g., binary; and ternary) systems, using the system’s physicochemical characteristics as inputs. Based on a database comprising hydration kinetics profiles of 235 unique systems—encompassing 7 synthetic cements and three mineral additives with disparate physicochemical attributes—a random forests (RF) model was rigorously trained to establish the underlying composition-reactivity correlations. This training was subsequently leveraged by the RF model: to predict time-dependent hydration kinetics of cement in new, multicomponent systems; and to formulate optimal mixture designs that satisfy user-imposed kinetics criteria.
Available at: http://works.bepress.com/jie-huang/175/
Financial support for this research was provided by the UM system; the Federal Highway Administration (Award No. 693JJ31950021); the Leonard Wood Institute (LWI: W911NF-07-2-0062) and the National Science Foundation (NSF-CMMI: 1661609 and 1932690).