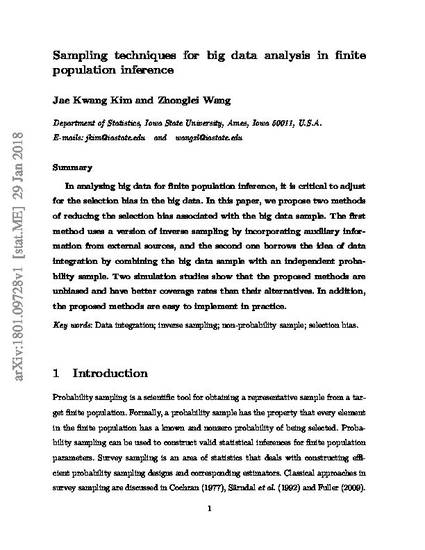
Article
Sampling Techniques for Big Data Analysis
International Statistical Review
Document Type
Article
Disciplines
Publication Version
Submitted Manuscript
Publication Date
5-1-2019
DOI
10.1111/insr.12290
Abstract
In analysing big data for finite population inference, it is critical to adjust for the selection bias in the big data. In this paper, we propose two methods of reducing the selection bias associated with the big data sample. The first method uses a version of inverse sampling by incorporating auxiliary information from external sources, and the second one borrows the idea of data integration by combining the big data sample with an independent probability sample. Two simulation studies show that the proposed methods are unbiased and have better coverage rates than their alternatives. In addition, the proposed methods are easy to implement in practice.
Copyright Owner
The Authors. International Statistical Review. International Statistical Institute
Copyright Date
2018
Language
en
File Format
application/pdf
Citation Information
Jae Kwang Kim and Zhonglei Wang. "Sampling Techniques for Big Data Analysis" International Statistical Review Vol. 87 Iss. S1 (2019) p. S177 - S191 Available at: http://works.bepress.com/jae-kwang-kim/58/
This is a manuscript of an article published as J.K. Kim and Z. Wang (2019). "Sampling Techniques for Big Data Analysis," International Statistical Review, 87, S177-S191. doi: 10.1111/insr.12290. Posted with permission.