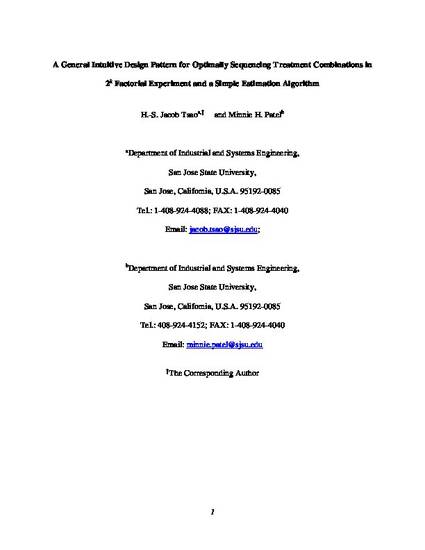
Article
A general intuitive design pattern for optimally sequencing treatment combinations in 2k factorial experiment and a simple estimation algorithm
Computers & Industrial Engineering
(2015)
Abstract
The number of model parameters of a 2k factorial design grows exponentially. When the number of factors is large, numerous higher-order interactions constitute a vast majority of the model parameters while many of them do not exist or are insignificant. The classic methods of fractional factorial designs, Plackett–Burman designs, Taguchi designs, etc. seek an already developed and often cataloged design that fits exactly the problem being tackled or select a design that fits it the most. Most, if not all, of these designs were developed in absence of convenient computation tools and enjoy computational simplicity. The necessary number of treatment combinations for unbiased estimation of significant parameters is often exceeded; undesirable confounding, i.e., biased estimation, is difficult to avoid. An opposite approach is to determine, for any set of model parameters considered as significant, a corresponding set of equal (and minimum) number of treatment combinations for unbiased parameter estimation. A companion feature of that approach is active avoidance of confounding. In addition, if the experimenter, particularly when unsure of the “borderline” significance of some parameters, can attempt to sequence model parameters in non-increasing order of magnitude (based on prior knowledge or subjective judgment), a corresponding sequence of treatment combinations can be numerically determined (with one treatment combination added for each additional possibly significant model parameter). Recently, a simple design pattern was proposed with which such a sequence of treatment combinations can be intuitively and easily obtained, without numerical computation. However, that pattern requires for the estimability of an interaction that all main effects and all lower-order interactions among all factors involved in the experiment have been estimated. For example, the interaction AB may not be estimable without main effect C having been estimated first. This paper relaxes that requirement and extends the use condition of the proposed approach to virtually all practical situations and presents a simple algorithm to estimate model parameters recursively. As sequential experimentation progresses, no experiments already conducted could be considered unnecessary for unbiased estimation of significant parameters, and hence “forward compatibility” in minimizing the number of treatment combinations is achieved. Therefore, such optimality may be referred to as FC-optimality or, more intuitively, as optimality of ‘one more treatment combination for one more parameter’ and ‘1-1-Optimality’ for short.
Keywords
- Design of experiment,
- Two-level factorial design,
- Fractional factorial design,
- Run minimization,
- Parameter estimability,
- Sequential design
Publication Date
2015
DOI
10.1016/j.cie.2015.04.001
Publisher Statement
This is the Author's Original Manuscript of an article that appeared in Computers & Industrial Engineering, 85, 2015. The Version of Record is available at the following link: http://doi.org/10.1016/j.cie.2015.04.001.
SJSU users: use the following link to login and access the article via SJSU databases.
Citation Information
H.-S. Jacob Tsao and Minnie H. Patel. "A general intuitive design pattern for optimally sequencing treatment combinations in 2k factorial experiment and a simple estimation algorithm" Computers & Industrial Engineering Vol. 85 (2015) p. 423 - 436 ISSN: 0360-8352 Available at: http://works.bepress.com/jacob_tsao/104/