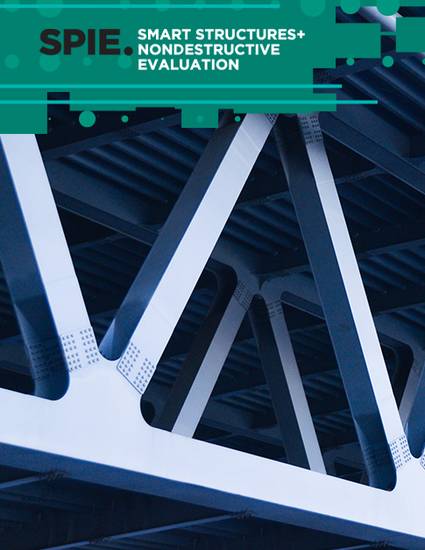
Article
An algorithmic framework employing tensor decomposition and Bayesian inference for data reconstruction in intelligent transportation systems
Nondestructive Characterization and Monitoring of Advanced Materials, Aerospace, Civil Infrastructure, and Transportation XV
(2021)
Abstract
Intelligent transportation systems (ITS) collect traffic data from various sensors deployed in smart cities. Yet, such information that are ubiquitous in real-world transportation systems mainly suffer from irregular spatial and temporal resolution. Consequently, missing and incomplete traffic data are inevitable as a result of detector and communication malfunctions when collecting information from ITS. Reconstructing missing values is then of great importance yet challenging due to difficulties in capturing spatiotemporal traffic patterns. This paper presents a transportation data reconstruction/imputation model that leverages Bayesian inference and tensor decomposition/completion to effectively address the missing data problem. On this basis, Bayesian tensor train (TT) decomposition is incorporated with Markov chain Monte Carlo Gibbs sampler to learn parameters of the model. Results of the experiments indicate that the proposed Bayesian TT model can effectively impute missing traffic data with acceptable accuracy.
Disciplines
Publication Date
March, 2021
DOI
https://doi.org/10.1117/12.2591181
Citation Information
Hadi Salehi. "An algorithmic framework employing tensor decomposition and Bayesian inference for data reconstruction in intelligent transportation systems" Nondestructive Characterization and Monitoring of Advanced Materials, Aerospace, Civil Infrastructure, and Transportation XV (2021) Available at: http://works.bepress.com/hsalehi/6/