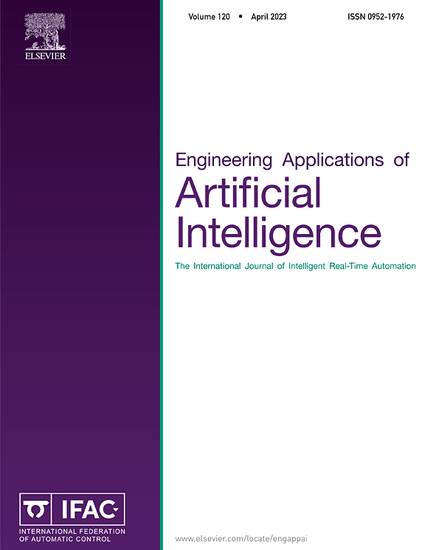
Article
Data interpretation framework integrating machine learning and pattern recognition for self-powered data-driven damage identification with harvested energy variations
Engineering Applications of Artificial Intelligence
(2019)
Abstract
Data mining methods have been widely used for structural health monitoring (SHM) and damage identification for analysis of continuous signals. Nonetheless, the applicability and effectiveness of these techniques cannot be guaranteed when dealing with discrete binary and incomplete/missing signals (i.e., not continuous in time). In this paper a novel data interpretation framework for SHM with noisy and incomplete signals, using a through-substrate self-powered sensing technology, is presented within the context of artificial intelligence (AI). AI methods, namely, machine learning and pattern recognition, were integrated within the data interpretation framework developed for use in a practical engineering problem: data-driven SHM of plate-like structures. Finite element simulations on an aircraft stabilizer wing and experimental vibration tests on a dynamically loaded plate were conducted to validate the proposed framework. Machine learning algorithms, including support vector machine, k-nearest neighbor, and artificial neural networks, were integrated within the developed learning framework for performance assessment of the monitored structures. Different levels of harvested energy were considered to evaluate the robustness of the SHM system with respect to such variations. Results demonstrate that the SHM methodology employing the proposed machine learning-based data interpretation framework is efficient and robust for damage detection with incomplete and sparse/missing binary signals, overcoming the notable issue of energy availability for smart damage identification platforms being used in structural/infrastructure and aerospace health monitoring. The present study aims to advance data mining and interpretation techniques in the SHM domain, promoting the practical application of machine learning and pattern recognition with incomplete and missing/sparse signals in smart cities and smart infrastructure monitoring.
Disciplines
Publication Date
November, 2019
DOI
https://doi.org/10.1016/j.engappai.2019.08.004
Citation Information
Hadi Salehi, Subir Biswas and Rigoberto Burgueño. "Data interpretation framework integrating machine learning and pattern recognition for self-powered data-driven damage identification with harvested energy variations" Engineering Applications of Artificial Intelligence (2019) Available at: http://works.bepress.com/hsalehi/13/