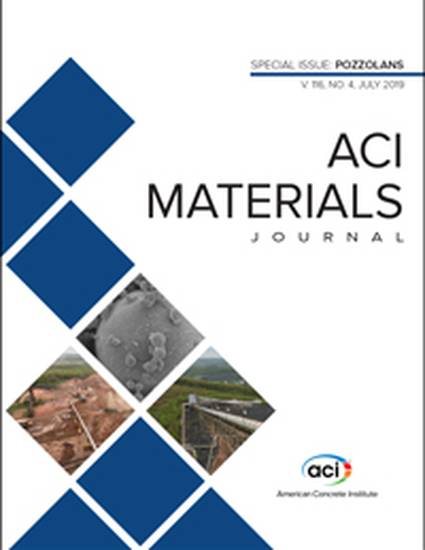
Article
Machine learning-driven assessment of fire-induced concrete spalling of columns
ACI Materials Journal
(2020)
Abstract
The past few years have witnessed the rise of serious research efforts directed toward understanding fire-induced spalling in concrete. Despite these efforts, one continues to fall short of arriving at a thorough examination of this phenomenon and of developing a modern assessment tool capable of predicting the occurrence and intensity of spalling. Unlike other works, this paper presents an approach that leverages a combination of machine learning (ML) techniques, namely k-nearest neighbor (k-NN) and genetic programming (GP), to examine spalling in fire-tested reinforced concrete (RC) columns. In this analysis, due diligence was taken to examine 11 factors known to influence spalling and to identify those of highest impact to be then used to develop a predictive tool. The outcome of this analysis shows that it is possible to predict the occurrence of spalling (with a successful rate ranging from 77 to 90%) through a simple, robust, and easy-to-use ML-driven tool.
Disciplines
Publication Date
November, 2020
DOI
10.14359/51728120
Citation Information
M Z Naser and Hadi Salehi. "Machine learning-driven assessment of fire-induced concrete spalling of columns" ACI Materials Journal (2020) Available at: http://works.bepress.com/hsalehi/10/