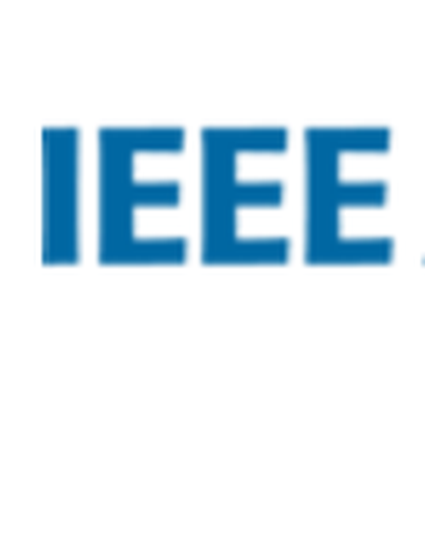
Distributed Bayesian inference provides a full quantification of uncertainty offering numerous advantages over point estimates that autonomous sensor networks are able to exploit. However, fully-decentralized Bayesian inference often requires large communication overheads and low network latency, resources that are not typically available in practical applications. In this paper, we propose a decentralized Bayesian inference approach based on stochastic gradient Langevin dynamics, which produces full posterior distributions at each of the nodes with significantly lower communication overhead. We provide analytical results on convergence of the proposed distributed algorithm to the centralized posterior, under typical network constraints. We also provide extensive simulation results to demonstrate the validity of the proposed approach.